質的研究:SCAT分析専用API-qualitative analysis with SCAT method
AI-powered tool for structured qualitative analysis

Related Tools
Load More
用户心理打标
分析文案,识别社会心理学现象

【就活/転職】業界分析・企業分析GPT
指定した業界や企業について、対話を通じて様々な情報を提供します。
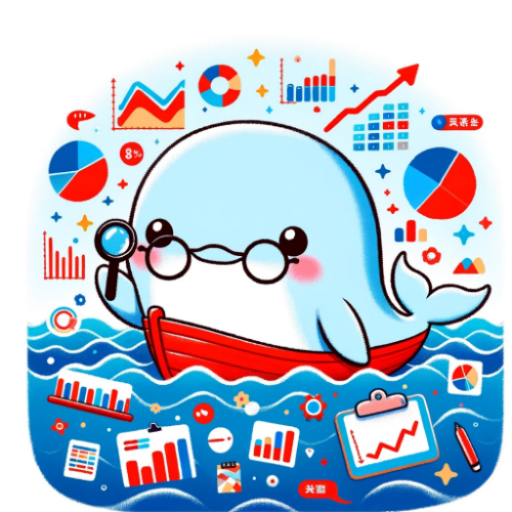
マーケ調査するぞ!
サービス特徴、3C分析、重点施策とスケジュールなどをアウトプットします。始めるには「開始する」を選択してください。

Quali Research - Text analysis Assistant
Expert in qualitative text analysis.

AI 선행연구 조사
연구주제를 잡기 위해 선행연구를 조사하고 동향을 파악하며 한계를 발견합니다.

落合フォーマット
Analyzes papers in a specified format
20.0 / 5 (200 votes)
Introduction to 質的研究: SCAT分析専用API
The 質的研究: SCAT分析専用API is designed to support researchers in performing qualitative research analysis using the SCAT (Steps for Coding and Theorization) method. SCAT is a structured coding and theorization method, particularly suited for small-scale qualitative data. It provides a clear, step-by-step procedure for analyzing qualitative data such as interview transcripts or open-ended survey responses, helping to derive meaningful concepts and theories. The API facilitates the application of SCAT by automating parts of this process, making qualitative data analysis accessible, especially to those who are more accustomed to quantitative methods. SCAT divides the analysis into four steps: (1) extracting notable phrases from the data, (2) rephrasing these using external terms, (3) explaining them in context, and (4) deriving themes or concepts. These themes are then woven into a coherent 'storyline' that supports theory development. A typical scenario is analyzing interview transcripts in the education sector, where SCAT helps to break down and systematically analyze responses to uncover underlying themes, making theory formation more manageable.
Main Functions of SCAT分析専用API
Automated Data Segmentation
Example
The API automatically segments qualitative data, such as interview transcripts, into smaller, manageable units for analysis.
Scenario
A researcher conducting a series of interviews on student experiences can use the API to break down the transcripts into relevant segments, ready for coding.
4-Step Coding Process
Example
The API guides the user through the four coding steps of SCAT—identifying key phrases, rephrasing with external terms, explaining context, and deriving themes.
Scenario
In a study on patient care, the API helps researchers pinpoint key observations in interview data, rephrase them, and construct meaningful themes about patient-provider interactions.
Theory Building Assistance
Example
The API assists in linking the derived themes into a coherent storyline and theory, providing suggestions for further conceptual development.
Scenario
An education researcher investigating teaching methods can use the API to generate a storyline from the data, leading to a new theory on teacher-student engagement.
Ideal Users of SCAT分析専用API
Qualitative Researchers
Researchers in fields such as education, sociology, psychology, and healthcare who rely on qualitative data to explore complex, human-centered phenomena. These users benefit from the structured, step-by-step process of SCAT to make sense of small-scale data and uncover underlying patterns.
Quantitative Researchers Transitioning to Qualitative Methods
Researchers with a background in quantitative methods who want to explore qualitative research. SCAT’s clear and systematic process reduces the complexity of qualitative analysis, making it approachable for those used to quantitative analysis frameworks, especially in fields like medical research and education.
How to Use 質的研究:SCAT分析専用API
Visit aichatonline.org for a free trial
No login or ChatGPT Plus subscription is required for the trial.
Input your qualitative data
Begin by pasting or uploading your interview transcripts or observational notes directly into the interface.
Select coding options
Choose the type of coding: data phrases, rephrased concepts, explanations, and emerging themes using the SCAT method.
Generate analysis
The API will process the codes and automatically generate a storyline and theory based on SCAT's structured steps.
Review and export results
You can refine the results, review the codes and theoretical insights, and export them for further research or reporting.
Try other advanced and practical GPTs
WP Interpreter
AI-Powered Support for WordPress Success

Internal Audit and Risk Management GPT
AI-Enhanced Internal Audit and Risk Management

Character Creator: Character Sheet
AI-powered tool for detailed character creation.

Character Creator: Visual Identity
AI-powered detailed character creation.

Slogan
AI-powered slogans in seconds.
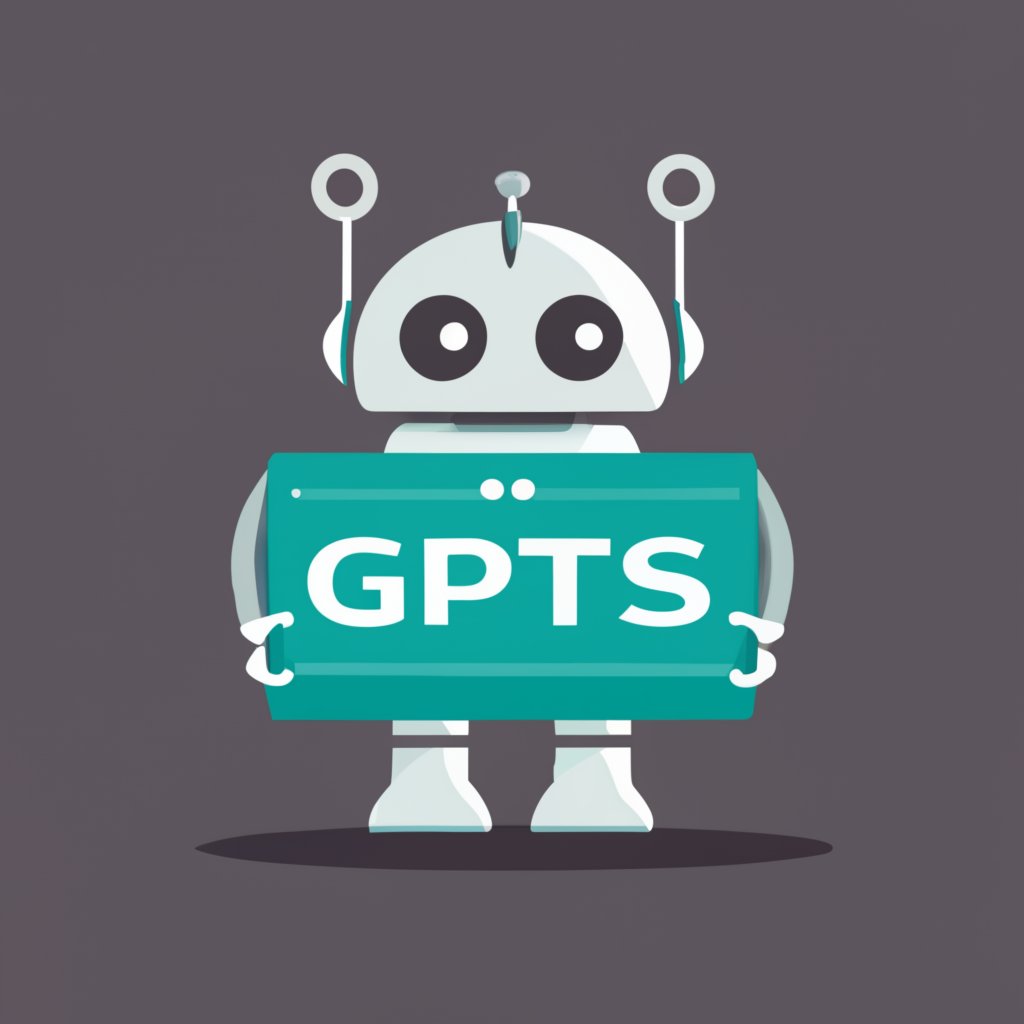
UX Copy Bot
AI-powered UX copywriting for seamless user experiences

Free-Hue Guide
AI-powered color technology at your fingertips

Laravel Expert 10.x for Japanese
AI-powered Laravel guidance for Japanese developers

繁譯家
AI-powered translation for Traditional Chinese.

Y Combinator Startup Assistant
AI-Powered Guidance for Startups
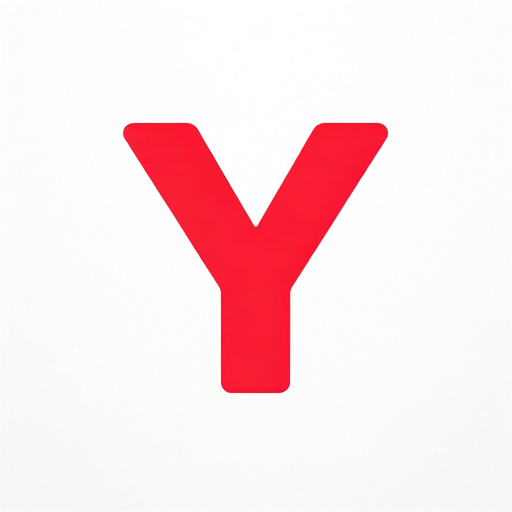
Machan AI
AI-powered conversations with a cultural twist.

GPT Crafter
AI-powered custom prompt creator.

- Research Papers
- Case Studies
- Survey Analysis
- Field Interviews
- Qualitative Insights
FAQs on 質的研究:SCAT分析専用API
What is the main purpose of 質的研究:SCAT分析専用API?
This API aids in qualitative data analysis using SCAT, a method designed to systematically analyze small-scale data, such as interviews and field notes, by coding and theorizing.
Who is SCAT analysis most suitable for?
SCAT analysis is ideal for qualitative researchers, especially those analyzing small-scale data, including beginners looking for clear and explicit procedures.
Can SCAT handle large datasets?
SCAT is optimized for small, qualitative datasets like individual interviews or open-ended survey responses. For larger datasets, more comprehensive methods like grounded theory may be preferable.
How does SCAT analysis differ from grounded theory?
While grounded theory focuses on broader, iterative data collection and theorization, SCAT provides a step-by-step, explicit process better suited for small-scale, qualitative research.
Is prior knowledge of qualitative research needed to use the API?
No advanced knowledge is required. The SCAT API provides a structured and guided approach, making it easy for beginners to apply qualitative analysis techniques effectively.