FAIR-AI-powered FAIR data compliance tool
AI-powered tool for making data FAIR

Create documentation for my data
My dataset is uploaded. Make it FAIR
Review my metadata
Write a DMP for my metadata
Assess data at URL.
Review my metadata
Related Tools
Load MoreActuarial Science
An expert in actuarial science, focusing on risk management and analysis.

▶️🏛️RELATÓRIO⚖️🔍
Um GPT com o único propósito de produzir um relatório detalhado e completo da petição inicial, contestação e réplica em casos complexos

⚖️ FIRAC+: ANÁLISE JURÍDICA PROFUNDA🏛️
Anexe as peças processuais relevantes e aperte ENTER. O FIRAC+ irá fazer a análise do seu caso

Good Faith Guardian
I'm here to elevate the quality of discussions by analyzing engagement, promoting good faith, and guiding debates toward constructive and respectful dialogue, while also offering insights into effective argumentation productive engagement.

Free-Equal
Especialista em Análise de Direitos Humanos em Contextos Multilíngues
Assessment Methodology
Please enhance the trustworthiness of this text, reword it, and adapt it for “keyword”.
20.0 / 5 (200 votes)
Introduction to FAIR
FAIR, which stands for Findable, Accessible, Interoperable, and Reusable, is a set of guiding principles designed to ensure that research data and its associated metadata can be easily located, understood, and utilized by both humans and machines. These principles support effective data management and stewardship, promoting data discovery and reuse across various disciplines. For instance, if a researcher publishes a dataset with clear, standardized metadata (e.g., using Dublin Core), it becomes discoverable in search engines like Google Dataset Search, reusable by others, and easy to integrate into new studies. FAIR practices are often required by major funding bodies such as the European Commission in programs like Horizon 2020, which encourages research projects to follow these principles to maximize the impact of their data.
Main Functions of FAIR
FAIRness Assessment
Example
A researcher can use FAIR evaluation tools such as FAIR enough or the France BioInformatique FAIR checker to measure how well their dataset adheres to FAIR principles.
Scenario
A university's research data management team assesses the FAIRness of their newly generated genomic dataset to ensure it's both findable and interoperable across genomic databases.
Metadata Review and Enrichment
Example
FAIR experts review metadata for completeness and suggest improvements like including persistent identifiers, standardized vocabularies, and ORCID for author identification.
Scenario
A team in a climate science project receives guidance on enriching their dataset’s metadata by incorporating geolocation, standardized climate terms, and a Creative Commons license to increase accessibility and reuse.
Repository and Journal Recommendations
Example
FAIR services can recommend relevant repositories or journals based on a dataset's subject area.
Scenario
A researcher working in ecology receives a recommendation to publish in Earth System Science Data (ESSD) journal and deposit data in the Global Biodiversity Information Facility (GBIF) repository.
Ideal Users of FAIR Services
Academic Researchers
Researchers, especially those working in data-intensive fields like bioinformatics, climate science, and social sciences, benefit greatly from FAIR services. They can ensure their datasets are well-documented, reusable, and meet the requirements of funding agencies or journals.
Research Data Managers and Data Stewards
Data managers are responsible for ensuring the data produced in research projects are compliant with institutional policies and FAIR principles. They can use FAIR services to assess datasets and improve the quality of metadata, thus ensuring long-term data reuse and interoperability.
How to Use FAIR: Step-by-Step Guide
Visit aichatonline.org for a free trial
Start by accessing aichatonline.org for a free trial. No login or subscription, such as ChatGPT Plus, is needed.
Understand the prerequisites
Ensure you have a basic understanding of research data, metadata, and data management concepts before diving into FAIR. Knowledge of common file formats and repositories is also useful.
Analyze data and metadata needs
Assess your dataset's structure, metadata quality, and readiness for FAIR principles: Findability, Accessibility, Interoperability, and Reusability.
Utilize FAIR APIs and repositories
Leverage APIs and tools like re3data, DataCite, and FAIR maturity tools for uploading, assessing, and managing your data according to FAIR standards.
Implement best practices and monitor updates
Continuously update data management plans (DMPs) and metadata as your project progresses, ensuring ongoing compliance with FAIR principles.
Try other advanced and practical GPTs
math
AI-powered math solutions at your fingertips
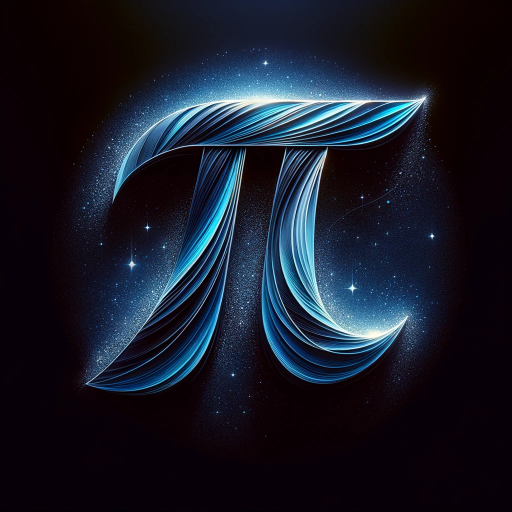
Détecteur d'IA
Detect AI-written content with ease.

Mandarin Mate
AI-powered translation for Chinese text

Seurat Specialist
AI-powered tool for Seurat analysis.

Image Editor - consistent generator
AI-Powered Precision Image Generation

visionOS Mentor for Apple Vision Pro
AI-powered mentor for spatial computing.

Image Match
Create webtoon art with AI precision

GPT News
AI-Powered News, In Your Hands.
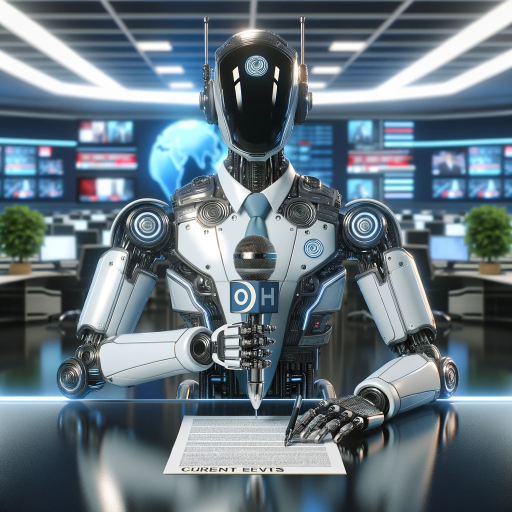
Legal Research AI
AI-Powered Legal Research Simplified

Pun Generator
AI-powered tool for creating witty puns
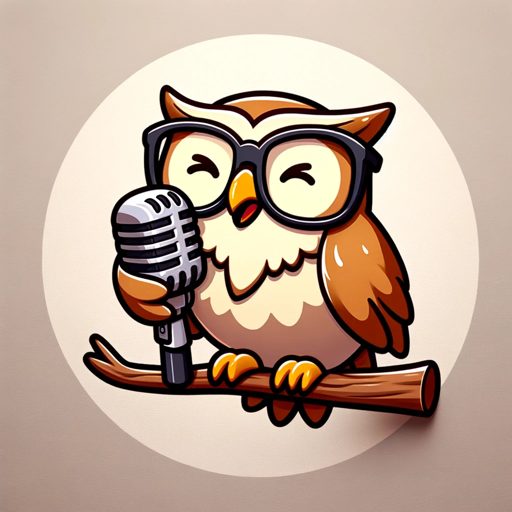
SNOMED Navigator
AI-powered SNOMED code generator

Visual Reconstructor
AI-powered image generation for precision.

- Metadata Creation
- Data Sharing
- FAIR Assessment
- Repository Upload
- DMP Writing
Frequently Asked Questions about FAIR
What does FAIR stand for?
FAIR stands for Findable, Accessible, Interoperable, and Reusable. These principles guide best practices for managing and sharing data in research.
Why is FAIR important?
FAIR ensures that research data is easily discoverable, accessible, and reusable by others, fostering transparency, collaboration, and innovation.
How can I make my data FAIR?
To make your data FAIR, provide detailed metadata, store it in a certified repository, apply proper licensing, and ensure it follows open data standards.
Which tools can help assess FAIR compliance?
Tools like the FAIR Data Maturity Model, re3data repository, and the FAIRsharing registry help assess and improve your dataset's FAIRness.
Is FAIR mandatory for Horizon 2020 projects?
Yes, under Horizon 2020, research data management must follow FAIR principles. However, opting out is possible if justified in the project’s data management plan (DMP).