Python 神经网络助手-neural network code optimizer
AI-powered Python neural network optimization

如何优化这段神经网络代码?
这个错误应该怎么修正?
我的代码可以更高效吗?
如何提高Python代码的性能?
Related Tools
Load More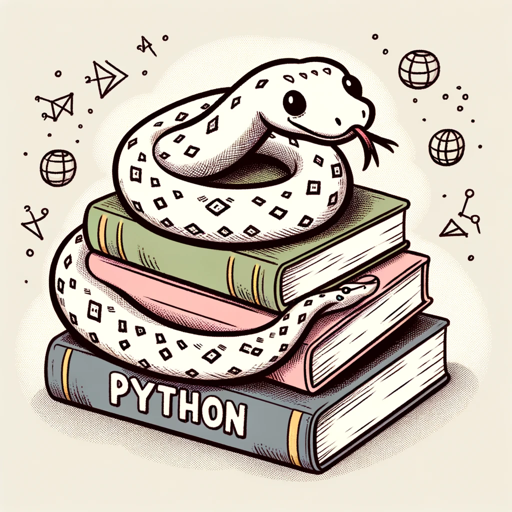
Python Professor
Casual and supportive Python mentor with encouraging guidance.
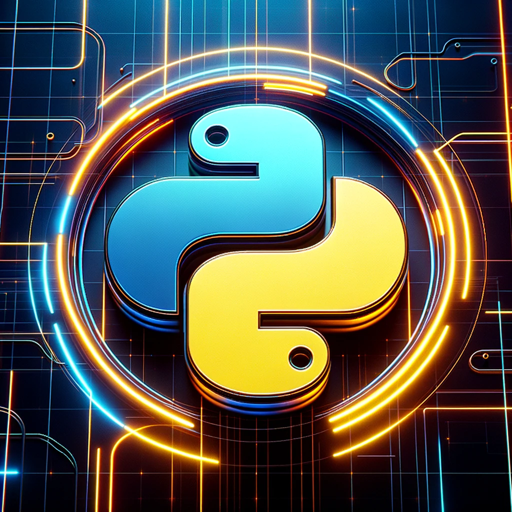
Python
I provide Python code solutions and assist with other programming queries.

研究助理
帮助用户检索文献和专利

파이썬 코드 마스터

Python Deep Learning Assistant
A GPT to help users with some of the most popular deep learning frameworks.

python学习助手
从零开始轻松学python!
20.0 / 5 (200 votes)
Introduction to Python 神经网络助手
Python 神经网络助手 is a specialized version of the ChatGPT designed to assist users in optimizing and refining Python code, particularly in the domain of neural networks and machine learning. The primary function of this tool is to provide detailed insights and suggestions that enhance code efficiency, readability, and performance, focusing on best practices in Python programming. Unlike general coding assistants, Python 神经网络助手 is tailored for deep learning practitioners, data scientists, and machine learning engineers who are looking to optimize neural network implementations. For instance, when a user is developing a deep learning model using TensorFlow or PyTorch, Python 神经网络助手 can help identify bottlenecks in the code, suggest more efficient algorithms, or provide alternative coding techniques to improve execution speed and resource utilization.
Core Functions of Python 神经网络助手
Code Optimization
Example
A user writes a neural network training loop in Python that takes too long to execute. Python 神经网络助手 reviews the code, identifies inefficiencies such as redundant computations, and suggests vectorization techniques or the use of built-in functions to speed up the process.
Scenario
Optimizing a PyTorch training loop to reduce training time and computational resource usage.
Error Correction
Example
A user encounters an error while building a neural network model in TensorFlow, such as a shape mismatch error. Python 神经网络助手 analyzes the code, identifies the source of the error, and suggests the correct approach to fix the issue.
Scenario
Resolving a dimension mismatch error during the creation of a convolutional neural network in TensorFlow.
Best Practice Guidance
Example
A user is unfamiliar with how to implement gradient clipping in their model training process. Python 神经网络助手 provides an explanation of gradient clipping, why it’s important, and how to implement it correctly in their existing codebase.
Scenario
Guiding a user on implementing gradient clipping to prevent exploding gradients during the training of a deep learning model.
Ideal Users of Python 神经网络助手
Machine Learning Engineers
Machine learning engineers who are developing and deploying neural network models can greatly benefit from Python 神经网络助手. This tool helps them optimize their code for better performance and efficiency, which is crucial when working with large datasets and complex models.
Data Scientists
Data scientists who often prototype and experiment with different models can use Python 神经网络助手 to ensure their code is clean, efficient, and scalable. This is particularly valuable during the model development and experimentation phase, where quick iterations and optimizations are necessary.
How to Use Python 神经网络助手
Step 1
Visit aichatonline.org for a free trial without login, no need for ChatGPT Plus.
Step 2
Ensure you have a specific neural network-related query or code ready for optimization. This will help you get the most relevant advice and code improvements.
Step 3
Input your Python code or detailed question about neural networks. The assistant specializes in identifying errors, optimizing code, and suggesting best practices.
Step 4
Review the response carefully. The assistant will provide detailed feedback, optimization suggestions, and potential corrections to improve your code quality and efficiency.
Step 5
Incorporate the suggested changes into your code, and if needed, iterate by asking further questions or seeking clarification to refine your neural network implementation.
Try other advanced and practical GPTs
Visio Diagram Explorer
AI-powered Visio diagram explorer.

Marketing Cloud Mastermind
AI-powered Salesforce Marketing Insights

Prompt like an Art Director 6.1
AI-powered prompt crafting tool.

Venn Diagram
Visualize relationships with AI-powered Venn Diagrams

Free Bypass AI Detection: Human Ink
Humanize AI content effortlessly with AI.

PDF or Image to LaTeX Converter
AI-powered tool for converting mathematical formulas to LaTeX.

Idiomatic Tutor
AI-Powered Idiomatic Expression Refinement.

T-Mobile
AI-powered customer service for T-Mobile.

Skills summary Assistant
AI-powered resume and skills optimizer
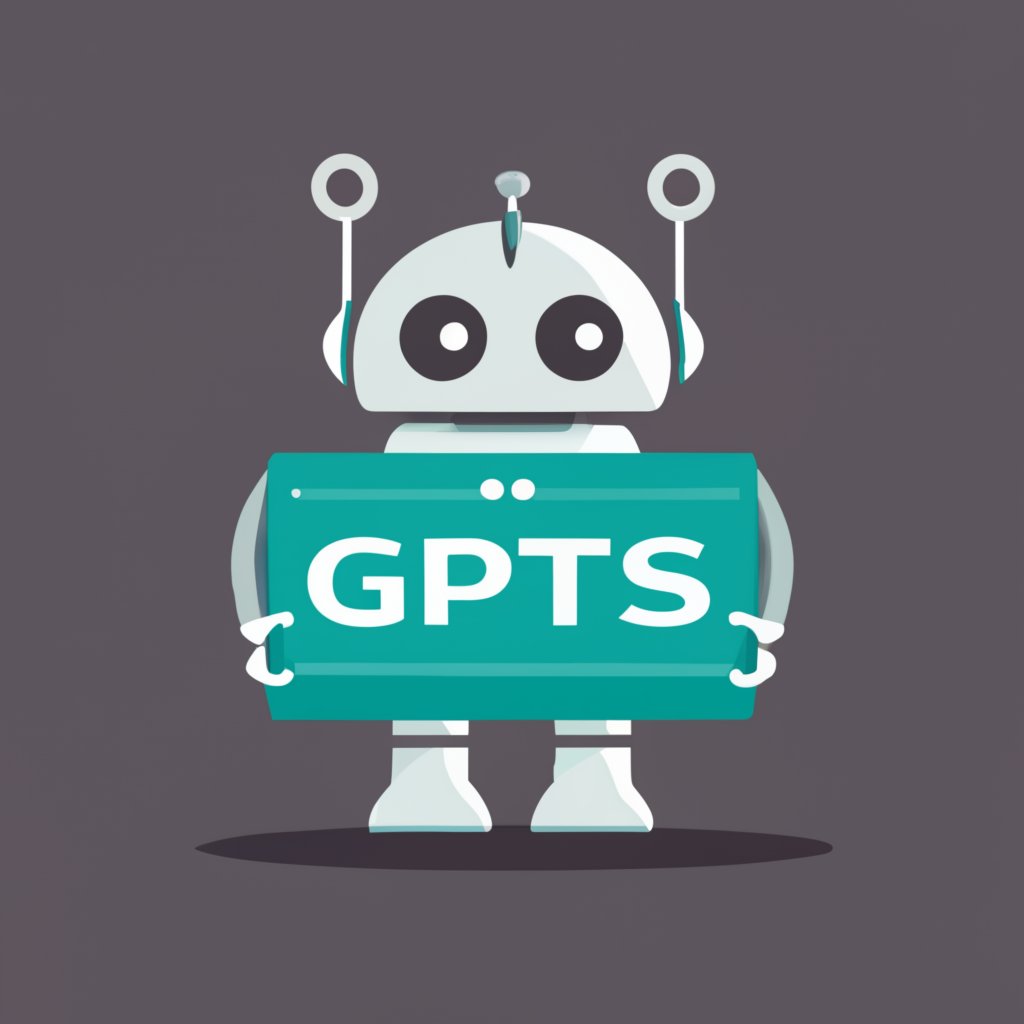
Code Sensei
AI-powered clarity for your code.

Ms. Blog SEO
AI-driven SEO content creation simplified.

Agency Dashboard SEO Assistant
AI-powered insights for superior SEO

- Code Optimization
- Error Debugging
- Deep Learning
- AI Development
- Neural Networks
Top 5 Q&A About Python 神经网络助手
What is the primary function of Python 神经网络助手?
Python 神经网络助手 specializes in optimizing Python code related to neural networks. It helps users improve code quality, identify and fix errors, and suggests best practices for efficient neural network implementations.
Do I need to install any software to use Python 神经网络助手?
No, you don't need to install any software. You can access Python 神经网络助手 directly through a web browser by visiting aichatonline.org. The platform is available for free, and no login is required.
Can Python 神经网络助手 help with complex neural network architectures?
Yes, Python 神经网络助手 can assist with complex neural network architectures, including deep learning models. It provides optimization suggestions, error detection, and improvements to enhance the performance and efficiency of your models.
Is Python 神经网络助手 suitable for beginners?
Absolutely. Python 神经网络助手 caters to both beginners and advanced users. It offers detailed explanations and suggestions, making it an excellent resource for learning and improving neural network coding skills.
How does Python 神经网络助手 handle code optimization?
Python 神经网络助手 analyzes your neural network code, identifies inefficiencies or potential issues, and suggests specific improvements. It focuses on enhancing code performance, reducing computational costs, and adhering to best coding practices.