Databricks-AI-powered analytics and collaboration
AI-powered data analysis made simple

Explain a Databricks concept
Guide me through a Databricks feature
Best practices in Databricks
Clarify a Databricks query
Related Tools
Load More
Pyspark Data Engineer
Technical Data Engineer for PySpark , Databricks and Python

Dashboard
Chatbot specialized in data analysis and dashboards with specific skills in SQL, Python, R, Excel, Tableau and Power BI

Data Dynamo
A friendly data science coach offering practical, useful, and accurate advice.

Azure Data Engineer
AI expert in diverse data technologies like T-SQL, Python, and Azure, offering solutions for all data engineering needs.
Databricks GTP

chatdbt
dbt developer assistant
20.0 / 5 (200 votes)
Introduction to Databricks
Databricks is a unified analytics platform designed to enhance data engineering, machine learning, and business analytics workflows. Its core purpose is to streamline data processes, making it easier to build, manage, and scale big data and AI-driven applications. The platform is built on Apache Spark, providing scalable, distributed data processing capabilities. What makes Databricks particularly powerful is its ability to integrate with cloud storage and data lakes (such as AWS S3, Azure Data Lake), allowing users to work with structured and unstructured data. It is designed for collaborative work environments, where data engineers, data scientists, and business analysts can work together seamlessly within the same workspace, sharing insights and models. A common scenario illustrating Databricks’ functionality is a retail company needing to analyze vast amounts of customer transaction data to optimize its marketing strategies. Using Databricks, the company can ingest large datasets from its cloud storage, clean and process the data using Apache Spark, then apply machine learning models to predict customer behavior, all within a single unified platform. With built-in notebooks and collaborative features, data scientists and analysts can co-develop these models, while data engineers ensure the infrastructure scales with increasing data volume.
Key Functions of Databricks
Unified Data Analytics
Example
A financial institution might need to process real-time transaction data to detect fraud patterns.
Scenario
Using Databricks, the institution can ingest and process large streams of data from multiple sources, apply complex algorithms to detect anomalies in real-time, and update models dynamically as new data becomes available. This unified approach to big data processing and analytics helps the institution detect fraud faster, saving both time and financial resources.
Collaborative Notebooks
Example
A data science team working on customer churn models can collaborate through shared notebooks in Databricks.
Scenario
Each team member can contribute code, data visualizations, and comments within the same notebook. Data engineers handle the data pipeline setup, data scientists experiment with machine learning algorithms, and business analysts can view results and provide feedback in real-time, fostering better collaboration and faster iteration on models.
Machine Learning & AI
Example
An e-commerce platform using Databricks for recommendation engines.
Scenario
By leveraging the machine learning libraries integrated with Databricks, such as MLlib, the platform can build models that analyze user behavior data (e.g., browsing history, past purchases) to recommend products. Databricks' scalable infrastructure enables continuous model retraining as new data flows in, improving the relevance of recommendations.
Ideal Users of Databricks
Data Engineers
Data engineers are responsible for building and maintaining scalable data pipelines. They benefit from Databricks' strong integration with cloud-based data lakes and scalable Apache Spark clusters, which makes it easier to ingest, transform, and optimize large datasets. By using Databricks, data engineers can develop complex data workflows without worrying about infrastructure management, thanks to its managed Spark environment.
Data Scientists
Data scientists use Databricks to experiment with data models and algorithms. They can easily access and process large datasets, leveraging built-in machine learning libraries and tools for fast prototyping. The collaborative environment in Databricks allows them to work more efficiently with other teams, while also scaling their machine learning models into production using the platform’s deployment features.
Guidelines for Using Databricks
Step 1
Visit aichatonline.org for a free trial without login, no need for ChatGPT Plus.
Step 2
Install any required integrations, such as connectors to cloud storage (e.g., AWS S3 or Azure Data Lake), to allow seamless data access and management.
Step 3
Familiarize yourself with the Databricks Workspace, which provides tools for managing notebooks, jobs, libraries, and clusters. Start by creating a cluster to begin running your data workloads.
Step 4
Explore the notebook environment for data processing, machine learning, or SQL-based analysis. You can write Python, Scala, SQL, or R code directly and interact with datasets from various sources.
Step 5
Leverage the collaborative features of Databricks, like sharing notebooks, working with teams on data projects, and using version control tools like Git to manage changes in your code.
Try other advanced and practical GPTs
Viral Singularity
AI-powered humor with no brakes.

PsychiatryPro AI
Enhance Your Mental Health Practice with AI

Academic Researcher Assistant
AI-Powered Research for Academia

Math Professor V2.0 (by GB)
AI-powered solutions for college math.

Personalized Fitness Trainer and Nutritionist
AI-Powered Fitness and Diet Planner

Cora the Corporate Controller
AI-powered financial accuracy and insight

Civil Engineering GPT
AI-Powered Civil Engineering Solutions

Intentional Eden
Empower your journey with AI-driven insights.
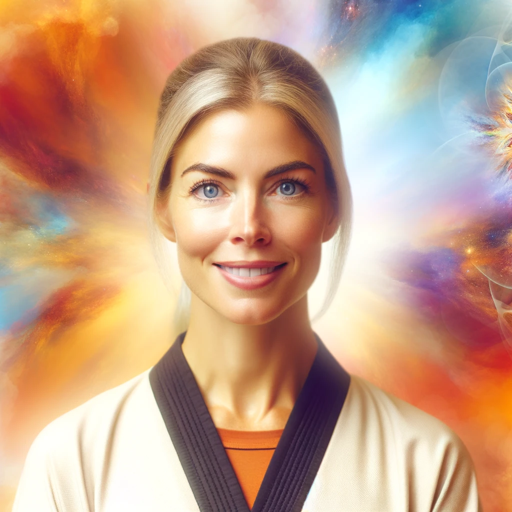
Dungeon Crawler
AI-powered dungeon crawling adventure.
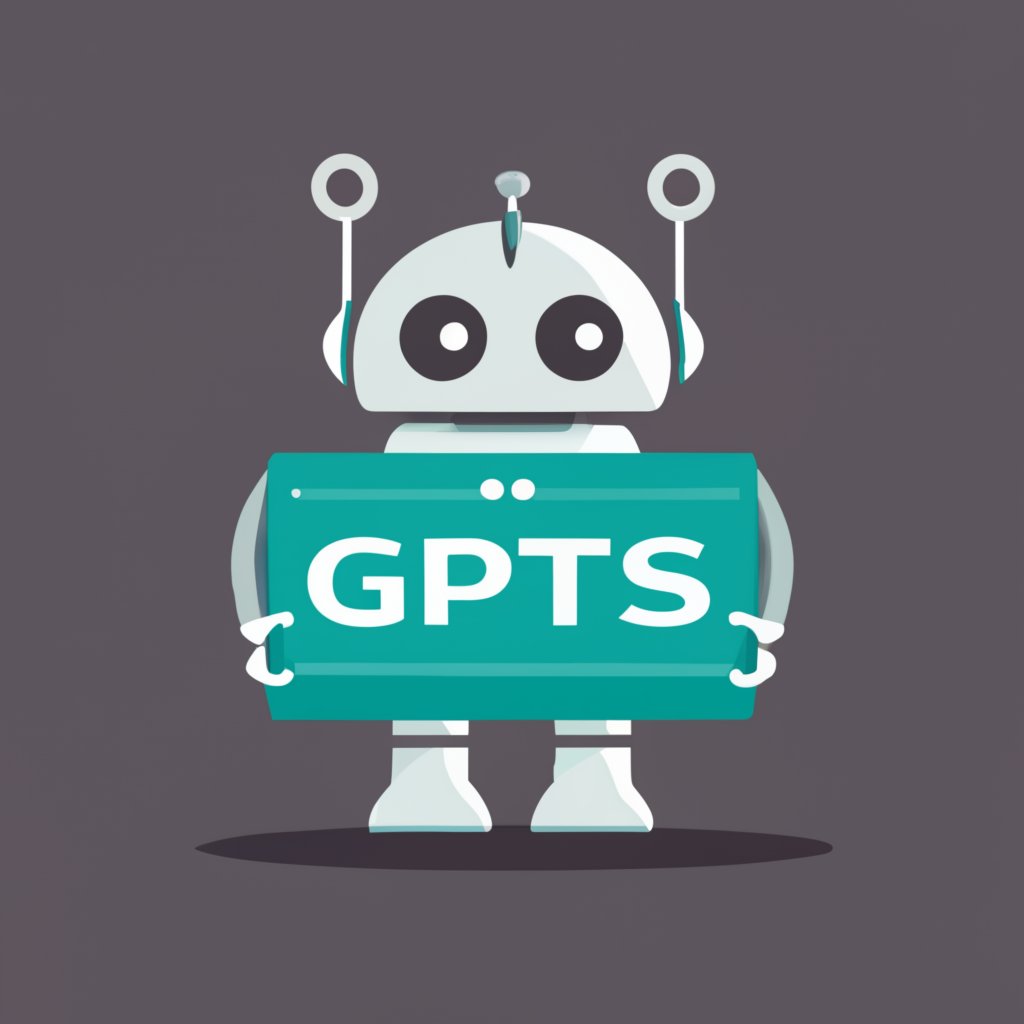
Image Upscaler by Mojju
AI-powered Image Upscaling Made Simple
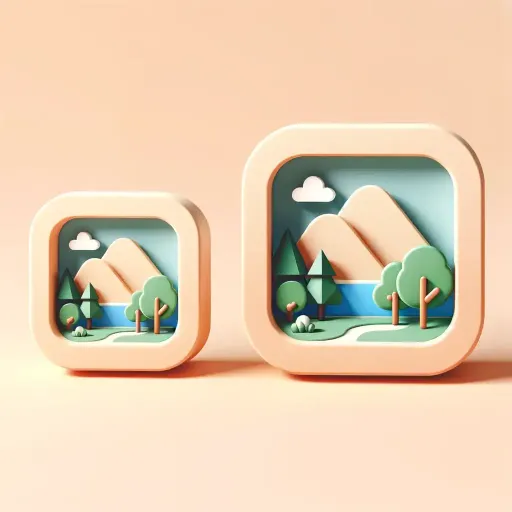
Themeco Pro Query GPT
AI-powered WordPress query generator.

Slide Wizard
AI-Enhanced Presentation Creation Tool
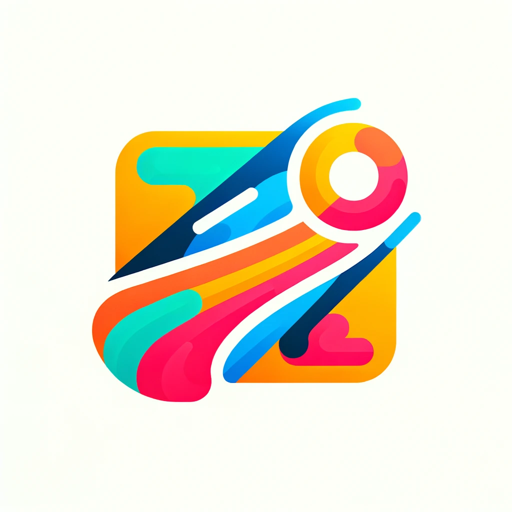
- Machine Learning
- Data Science
- Cloud Integration
- Big Data
- Data Engineering
Common Questions About Databricks
What is Databricks used for?
Databricks is a unified analytics platform that facilitates data engineering, machine learning, and business intelligence. It is commonly used for big data processing, advanced analytics, and collaborative development in cloud-based environments.
Can Databricks be used with different programming languages?
Yes, Databricks supports multiple languages, including Python, Scala, R, and SQL. This flexibility allows data scientists, analysts, and engineers to collaborate on various tasks using their preferred languages.
What is a Databricks cluster?
A Databricks cluster is a set of computing resources used to run data processing jobs or interactive notebooks. Clusters allow users to scale their computations and are an essential part of working efficiently with large datasets in Databricks.
How does Databricks integrate with cloud storage?
Databricks integrates seamlessly with major cloud platforms like AWS, Azure, and Google Cloud, allowing users to connect to cloud storage services such as S3, Azure Data Lake, or Google Cloud Storage for direct data processing.
What are the collaborative features of Databricks?
Databricks offers collaborative features like shared notebooks, real-time co-authoring, version control integration (e.g., Git), and the ability to track experiments and models, making it easy for teams to work together on data projects.