Image Context Tagger for LoRa Training-Image Context Captioning
AI-powered image context descriptions for precise LoRa training.

Related Tools
Load More
Image Locator
Analyzes images to identify locations, explains reasoning.
商品实体标注专家
following the instructions and Annotate
商品实体标注专家
following the instructions and Annotate

GeoGuesserGPT
Upload a picture and I'll analyze it better than any GeoGuesser Expert, crafting a clever guess to reveal where it was taken. Try GeoGuesserGPT and I'll pinpoint the spot with surprising insight!

Label Assistant
Lable the image/bulk images for model training
Training Material Design Advisor
Designs effective training materials to enhance organizational learning and performance.
20.0 / 5 (200 votes)
Introduction to Image Context Tagger for LoRa Training
Image Context Tagger for LoRa Training is designed to generate precise, factual descriptions of elements within an image, excluding the main subject, to aid in training LoRa models. This tool helps create detailed descriptions of the environment, background elements, secondary subjects, and specific details like objects, activities, and interactions. The purpose is to provide accurate and objective information without subjective language, focusing on clear, concrete terms. For example, if the main subject is a horse, the tagger would describe the lighting, background, and other elements around the horse without mentioning the horse itself. This ensures that the model learns to distinguish between the main subject and its context.
Main Functions of Image Context Tagger for LoRa Training
Detailed Background Description
Example
Describing the background of an image with a horse as the main subject, such as 'dark background with bokeh, blurry trees in the background, soft natural lighting, early evening light, shallow depth of field.'
Scenario
In a dataset of animal images, accurately describing the background helps the model learn to differentiate various environments animals might be in.
Environmental and Lighting Conditions
Example
Captions like 'warm sandstone hues, natural lighting, light from the side, 120mm f1.8' for an image of a black dog sitting at the entrance of a pyramid.
Scenario
For architectural or landscape datasets, detailing environmental and lighting conditions helps the model understand different lighting scenarios and their effects on images.
Describing Secondary Subjects
Example
In an anime drawing, noting elements like 'brown couch, red patterned fabric, wooden floor, refrigerator in background, coffee machine on a countertop, table in front of couch, bananas and coffee pot on table.'
Scenario
For scenes with multiple elements, providing details about secondary subjects ensures the model can accurately identify and distinguish various objects and their relationships within the scene.
Ideal Users of Image Context Tagger for LoRa Training
AI Model Trainers
Professionals developing and fine-tuning AI models, especially those working with image recognition and classification. They benefit from precise and consistent context tagging to improve model accuracy and robustness in identifying and differentiating between main subjects and their surroundings.
Dataset Curators
Individuals or teams responsible for creating and curating image datasets for training machine learning models. They use this tool to ensure high-quality, detailed descriptions of images, facilitating better training data and improving model performance.
How to Use Image Context Tagger for LoRa Training
1
Visit aichatonline.org for a free trial without login, no need for ChatGPT Plus.
2
Identify the main subject of your image to exclude it from the detailed description.
3
Use the tool to generate factual, objective descriptions of the environment, background, secondary subjects, and specific details in the image.
4
Review the generated captions to ensure they are consistent and avoid subjective language or repetition.
5
Apply these captions to your LoRa model training to enhance its ability to understand and generate accurate image contexts.
Try other advanced and practical GPTs
AI Sports Bet Picks
AI-Powered Predictions for Smarter Bets

Sports Picks by AiPicks.ai
AI-driven insights for smarter sports bets

Prize Picks Parlay Picker
AI-powered parlay and player prop picker.

Formal Document Draft Pro
AI-powered solution for formal documents

Multiple-Choice Quiz
AI-powered multiple-choice quizzes for language learners.
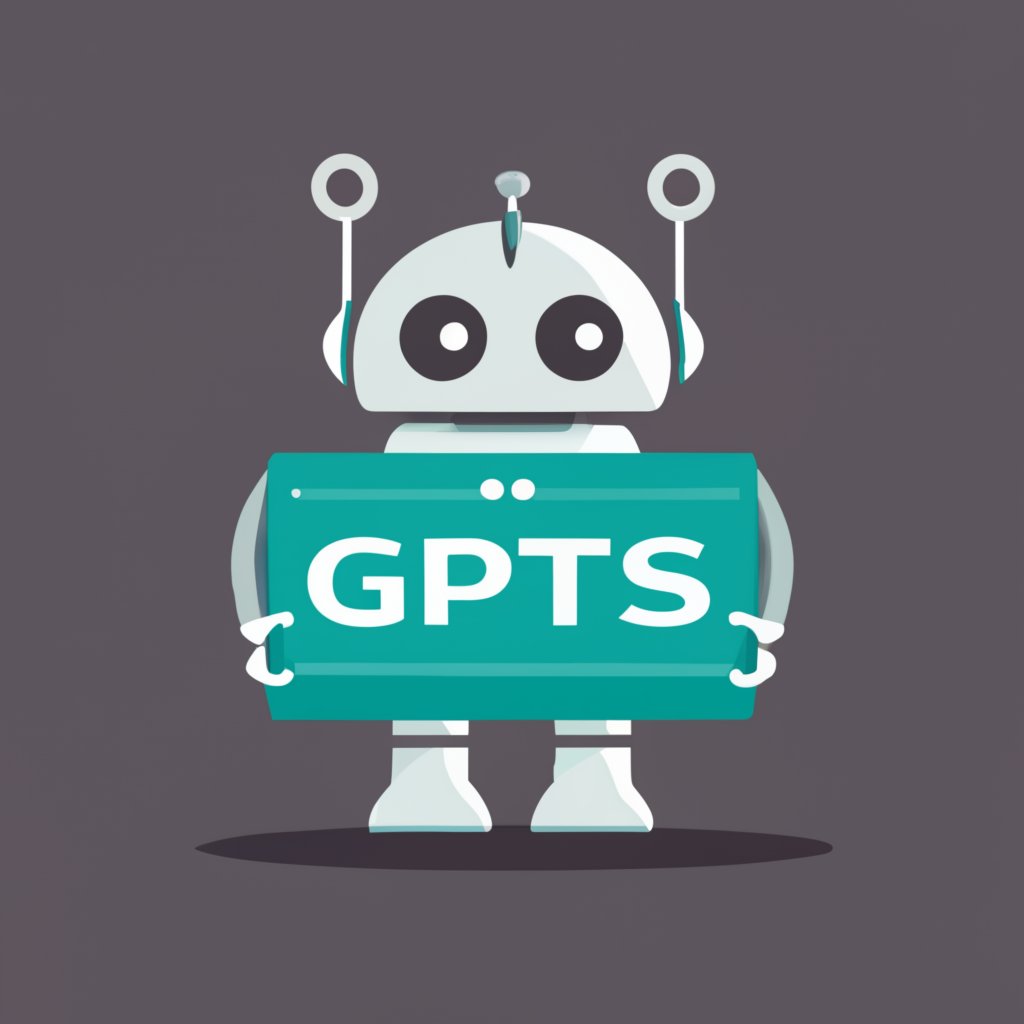
签到助手
AI-powered daily check-in tracking.

ChatPRD 👉🏼 With Diagrams
Enhance your PRDs with AI-powered diagrams.

HTML Master
AI-powered HTML newsletter creation

Web Design HTML Coder
Transform Your Designs into HTML Instantly

Story Telling
Craft compelling stories with AI

Story Quest
Unleash Your Imagination with AI-Powered Narratives

Coder Biliblippi
AI-powered coding help for every developer.

- Content Generation
- Model Training
- AI Training
- Image Captioning
- Data Annotation
Q&A about Image Context Tagger for LoRa Training
What is the main purpose of the Image Context Tagger for LoRa Training?
The main purpose is to provide precise and factual descriptions of all elements in an image, except the main subject, to improve the training of LoRa models.
How does the tool handle descriptions of the main subject?
The tool excludes the main subject from detailed descriptions and instead focuses on the environment, background, secondary subjects, and other specific details.
Can this tool be used for any type of image?
Yes, it can be used for various types of images, including photographs, illustrations, and drawings, provided the main subject is clearly identified.
What kind of language does the Image Context Tagger avoid?
The tool avoids subjective language, superlatives, and qualifiers, focusing instead on clear, objective descriptions.
What are some key benefits of using this tool for LoRa model training?
Key benefits include improved accuracy in image context understanding, consistency in training data, and enhanced model performance in generating and interpreting image descriptions.