Longitudinal and Survival Analysis-survival and longitudinal analysis tools
AI-powered survival and longitudinal analysis

Related Tools
Load More
Mathematical statistics
Expert in graduate-level mathematical statistics, providing detailed and accurate explanations.

Statistic & Data Analyst
Statistic and Data Analyst assistent
Actuarial Science
An expert in actuarial science, focusing on risk management and analysis.

医療統計GPT
医療統計GPTは、あなたの研究を支援するために、医療データ分析をサポートします。目標を明確にし、データを検証後、信頼できる統計方法で分析を行います。また使用された統計方法に関連する注意点、およびバイアスのリスクも合わせて提示します。このプロセスを通じ、データ駆動の意思決定を支援し、分析結果を直感的に理解しやすい形で表示します。日本語のグラフ表示にも対応しています。

R Code Sage
R coding expert minimizing errors in R programming

EpiStat - GPT
Advanced epidemiology concept specialist.
20.0 / 5 (200 votes)
Introduction to Longitudinal and Survival Analysis
Longitudinal and survival analysis are crucial statistical techniques designed to analyze different types of complex data. Longitudinal analysis focuses on repeated measurements of subjects over time, allowing researchers to understand changes in variables and relationships over a period. Survival analysis, or time-to-event analysis, is used to examine the time until a specific event occurs, such as death, disease recurrence, or device failure. The combination of these methods addresses scenarios where both continuous longitudinal data and discrete event data are present. For instance, a medical study might track a patient's biomarker levels over time while also recording the time until disease relapse. This approach allows for deeper insights by linking longitudinal markers to survival outcomes. These analyses are widely applied in clinical trials, reliability testing, and epidemiology to assess treatment efficacy, disease progression, or equipment durability.
Key Functions of Longitudinal and Survival Analysis
Linking Longitudinal Data to Survival Outcomes
Example
A study measuring a cancer patient's tumor size over time and predicting the time until remission or death.
Scenario
In cancer trials, continuous biomarker measures like tumor size, assessed at different points, can be modeled with longitudinal techniques while survival analysis predicts when the patient might relapse or die. Joint models allow for real-time updates of survival estimates as the tumor size changes.
Handling Time-Varying Covariates in Survival Models
Example
Monitoring blood pressure fluctuations to predict the likelihood of a heart attack in a patient cohort.
Scenario
In cardiovascular studies, time-varying covariates like blood pressure can be integrated into survival models to assess their dynamic impact on the time to a cardiac event. This allows continuous adjustments of risk predictions based on the latest data, improving intervention strategies.
Modeling Censored and Truncated Data
Example
A clinical study tracking patients' survival after surgery where some patients drop out or are lost to follow-up.
Scenario
In many studies, not all subjects experience the event of interest within the observation period. Survival analysis accounts for right-censoring (e.g., when a patient is lost to follow-up) and left-truncation (e.g., patients recruited after the study starts), ensuring unbiased estimates of survival probabilities.
Target Users for Longitudinal and Survival Analysis
Medical Researchers and Clinicians
Researchers in medicine and healthcare benefit from longitudinal and survival analysis to track disease progression, treatment efficacy, and predict patient outcomes. By integrating continuous health measurements with survival data, they can make real-time decisions, optimize treatment plans, and assess risk factors more accurately.
Epidemiologists and Public Health Analysts
Public health officials use these techniques to monitor and predict the spread of diseases and their impact on populations over time. Longitudinal data like infection rates can be linked to survival outcomes, such as death rates, allowing for effective policy interventions and resource allocation.
Using Longitudinal and Survival Analysis
Visit aichatonline.org for a free trial without login
Start by accessing the analysis tools available without the need for login or special software.
Prepare your data for analysis
Ensure you have both longitudinal data (repeated measures over time for each individual) and survival data (time-to-event). Ensure data is cleaned, especially handling missing values and censoring information for survival.
Choose the right model based on research questions
Decide between joint models, two-step approaches, or using time-dependent covariates based on whether you want to assess time-varying effects or jointly model longitudinal and time-to-event data【7†source】【8†source】.
Implement the model
Use software like SAS (PROC PHREG, NLMIXED, LIFEREG) or R (JM package) to apply either a proportional hazards model with time-varying covariates, or a joint model linking longitudinal outcomes to time-to-event【7†source】【9†source】.
Interpret and validate the results
Review estimates like hazard ratios or survival probabilities, check model assumptions (e.g., proportional hazards), and conduct sensitivity analyses to verify robustness of the findings【9†source】.
Try other advanced and practical GPTs
Research Linker
AI-powered tool for finding research articles

Medical Research
AI-Powered Insights for Medical Research

Research+
AI-Powered Comprehensive Research Solutions
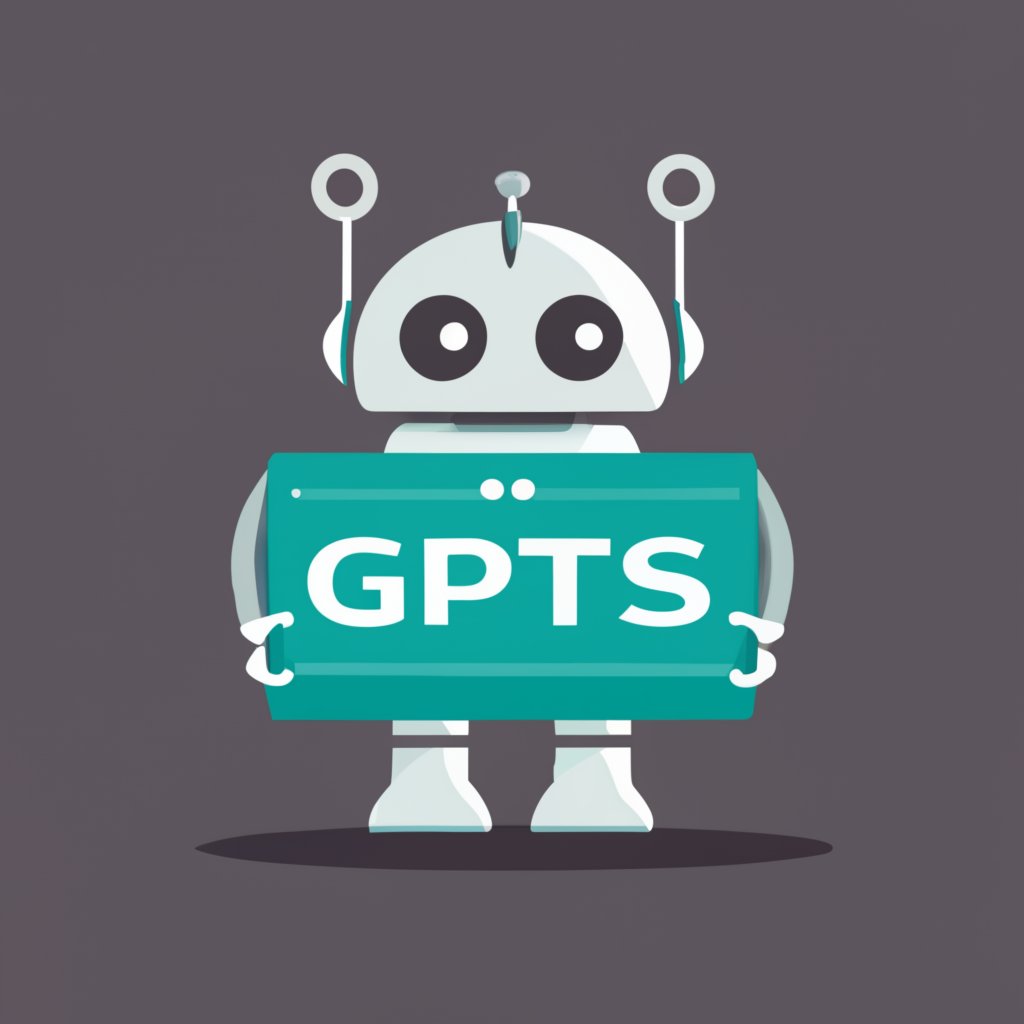
Domain Name Generator & Availability Checker
AI-Powered Domain Name Generator & Availability Checker
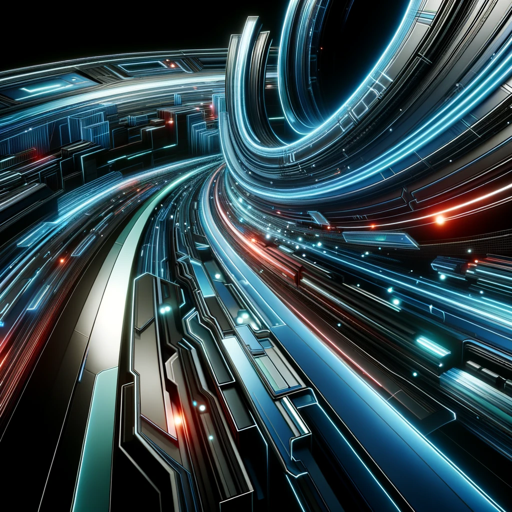
Document Scanner
AI-driven summaries for any document.

Document Translator
AI-powered Document Translation Made Easy

Organic Chemistry Tutor
AI-powered tool for mastering organic chemistry

Innovative Technology Consultant
AI-driven insights for technology strategy.

Image123【Refine+Variations】No More Prompt
Refine and transform images with AI.

Refine and Accurately Translate Email Responses
AI-Powered Email Refinement & Translation

Sentence Structure Bot - CE 101
AI-driven sentence structure enhancement.

Angry Email Translator
Turn angry emails into polite replies with AI.
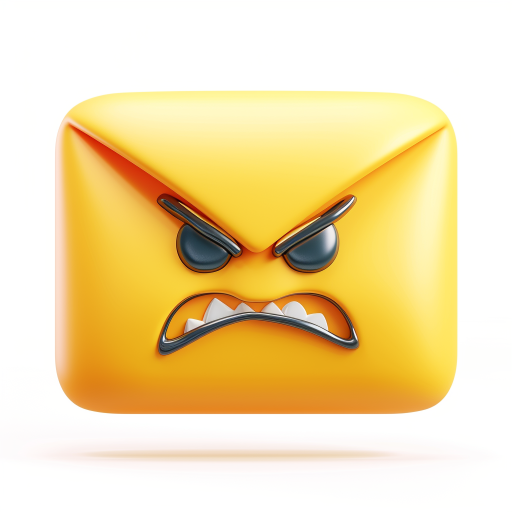
- Data Science
- Medical Research
- Clinical Trials
- Epidemiology
- Biostatistics
Q&A: Longitudinal and Survival Analysis
What is a joint model in survival analysis?
A joint model simultaneously analyzes longitudinal data (e.g., biomarker trajectories) and survival data (time-to-event), allowing for shared random effects and the assessment of how a time-varying covariate influences survival【7†source】【9†source】.
How does a two-step approach work for linking longitudinal and survival data?
First, a longitudinal model (e.g., linear mixed model) is fit to estimate trajectories, followed by a survival model using these estimated trajectories as covariates in the time-to-event analysis【7†source】.
What are time-dependent covariates in survival analysis?
These are variables whose values change over time and are used in survival models to adjust the hazard of the event occurring based on the covariate's value at different time points【8†source】.
What are the assumptions of the Cox proportional hazards model?
The main assumption is proportionality, meaning the hazard ratios between groups remain constant over time. This can be checked by examining the survival curves or statistical tests【9†source】.
What are some common challenges in analyzing longitudinal survival data?
Handling missing data, ensuring appropriate modeling of censored survival times, and accounting for time-varying effects or correlations in repeated measures are key challenges【8†source】【9†source】.