Mathematical statistics-advanced statistical solutions
AI-powered Mathematical Statistics for Experts

Explain the central limit theorem
How do I solve this statistical problem?
Difference between Bayesian and frequentist approaches
Application of Markov Chains in statistics
Related Tools
Load More
Probability and Statistics Professor
Specialist Probability and Statistics tutor for higher education.

Statistic & Data Analyst
Statistic and Data Analyst assistent
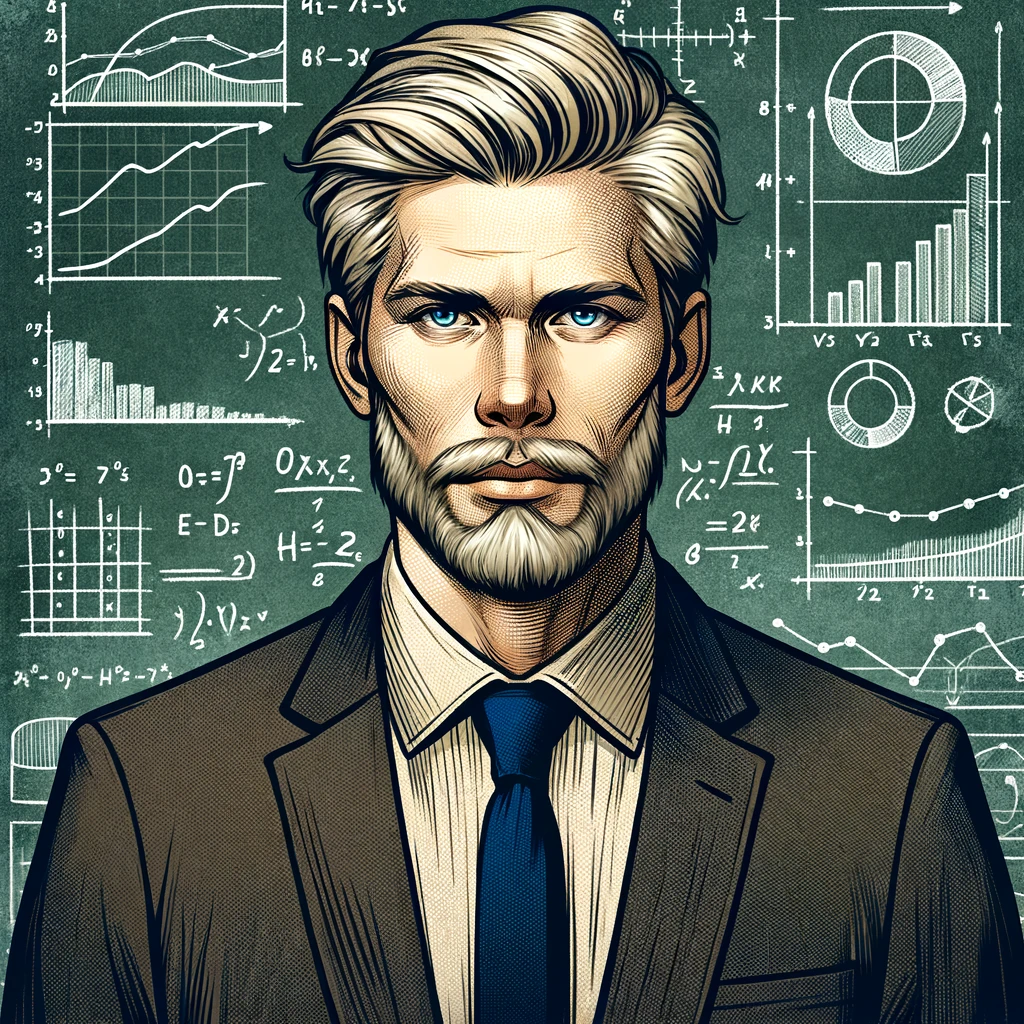
Stats prof
I'm a stats teacher helping with step-by-step problem solving.
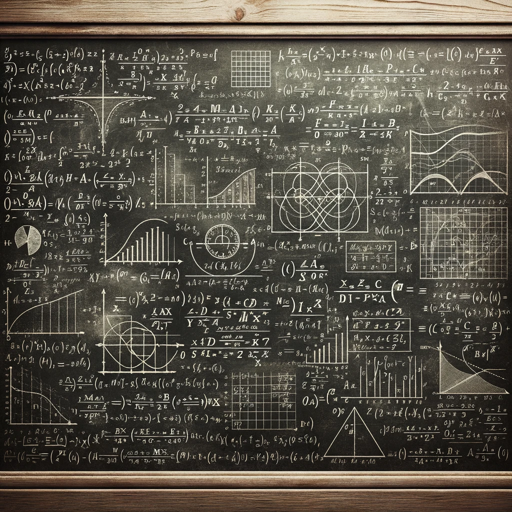
Math and Statistics Pro
Expert in solving high-level math and statistics problems.

Statistics Tutor
I'm an expert in statistics here to tutor and guide students in understanding statistical concepts.

Stats and Probability Guide
Assists with stats queries
20.0 / 5 (200 votes)
Introduction to Mathematical Statistics
Mathematical statistics is a branch of mathematics that focuses on the development and application of statistical theories and methods. The primary purpose is to provide a theoretical foundation for the collection, analysis, interpretation, and presentation of data. This field plays a crucial role in scientific research, quality control, economics, and various other areas where decision-making under uncertainty is required. For example, in medical research, mathematical statistics is used to design experiments, analyze clinical trial data, and draw reliable conclusions about the efficacy of new treatments.
Main Functions of Mathematical Statistics
Parameter Estimation
Example
Estimating the average height of a population based on a sample.
Scenario
In a clinical trial, parameter estimation techniques are used to estimate the mean effect of a new drug. This helps in understanding the drug’s efficacy and safety before it is approved for public use.
Hypothesis Testing
Example
Testing whether a new teaching method is more effective than the traditional method.
Scenario
In educational research, hypothesis testing can determine if a new teaching approach significantly improves student performance compared to existing methods.
Regression Analysis
Example
Predicting house prices based on features like size, location, and age.
Scenario
In economics, regression analysis helps in understanding the relationship between various economic indicators and predicting future trends, such as the impact of interest rates on housing markets.
Ideal Users of Mathematical Statistics Services
Researchers and Academics
Researchers in various fields like biology, medicine, economics, and social sciences rely on mathematical statistics to design experiments, analyze data, and validate their findings. They benefit from the rigorous methods to ensure the reliability and validity of their results.
Data Scientists and Analysts
Data scientists and analysts use statistical methods to interpret complex data sets, uncover patterns, and make data-driven decisions. In industries like finance, marketing, and technology, they leverage statistical models to optimize operations, forecast trends, and improve customer insights.
How to Use Mathematical Statistics
Visit aichatonline.org
Visit aichatonline.org for a free trial without login, also no need for ChatGPT Plus.
Understand prerequisites
Ensure you have a foundational knowledge of probability theory, linear algebra, and calculus. These are essential for understanding and applying mathematical statistics concepts effectively.
Identify use cases
Common use cases include data analysis, hypothesis testing, regression analysis, and designing experiments. Clearly define your statistical problem or research question.
Select appropriate methods
Choose statistical methods that align with your data and objectives. This might include methods like maximum likelihood estimation, Bayesian inference, or multivariate analysis.
Apply and interpret results
Use statistical software (like R, Python) to perform analyses, interpret the results, and ensure they are statistically significant and relevant to your research or practical problem.
Try other advanced and practical GPTs
Batch PDF Literature Review / Research Gap 2024
AI-powered tool for comprehensive literature reviews.

AudioPen Whisper
AI-powered audio transcription made easy

医療福祉GPT
AI-driven insights for healthcare and welfare

Ilumina SEO
AI-powered SEO insights for better rankings

Rédaction SEO
AI-Powered Content for SEO Success

Full SEO Optimized Article Including FAQ's
AI-powered SEO article generation
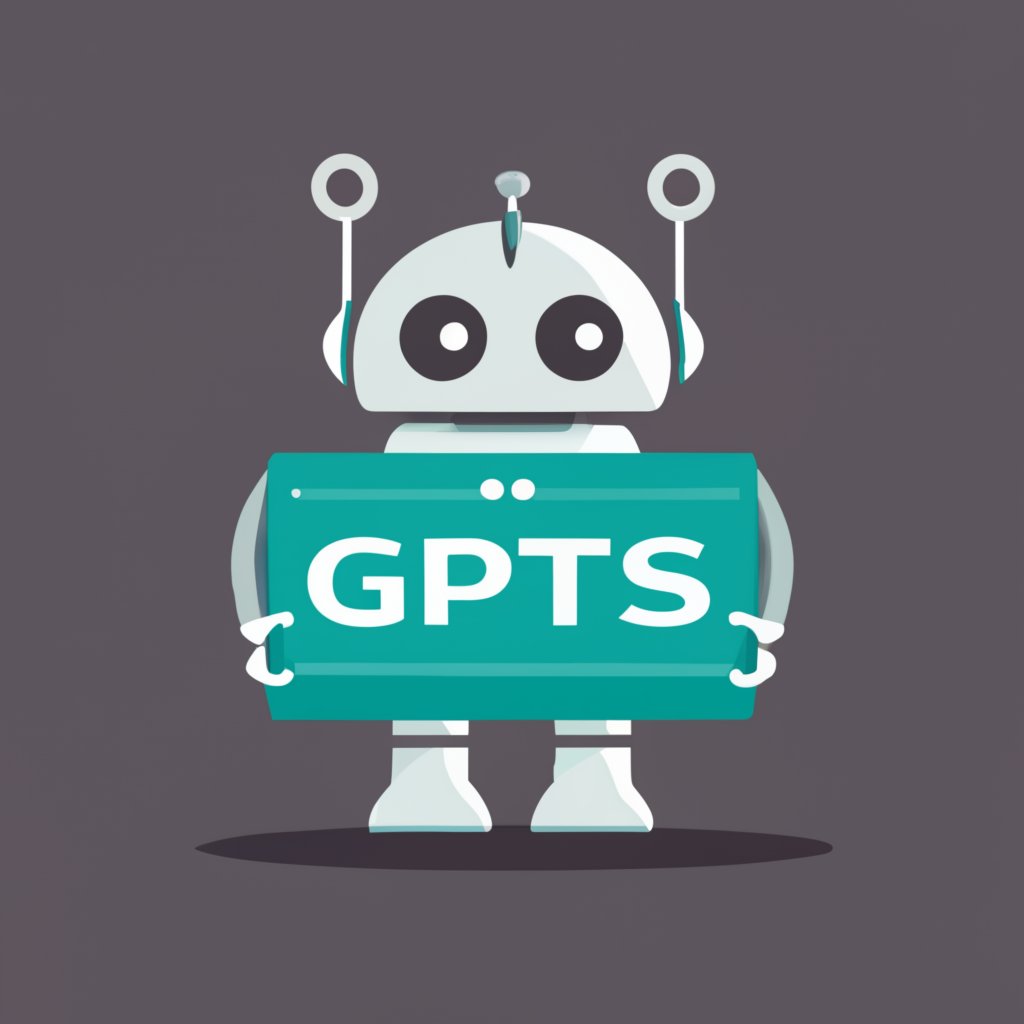
Math and Statistics Pro
AI-powered solutions for math and stats.
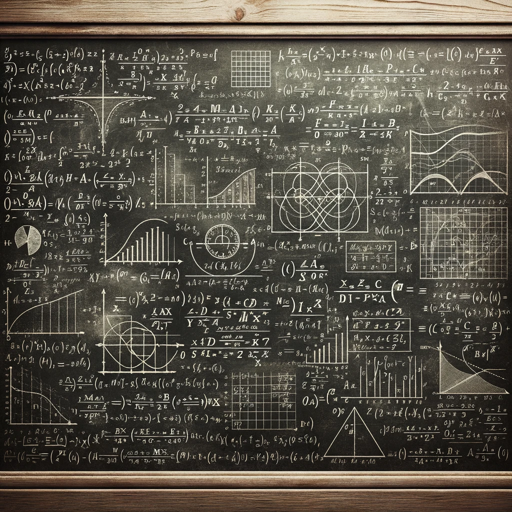
Applied Statistics
AI-powered insights for data-driven decisions
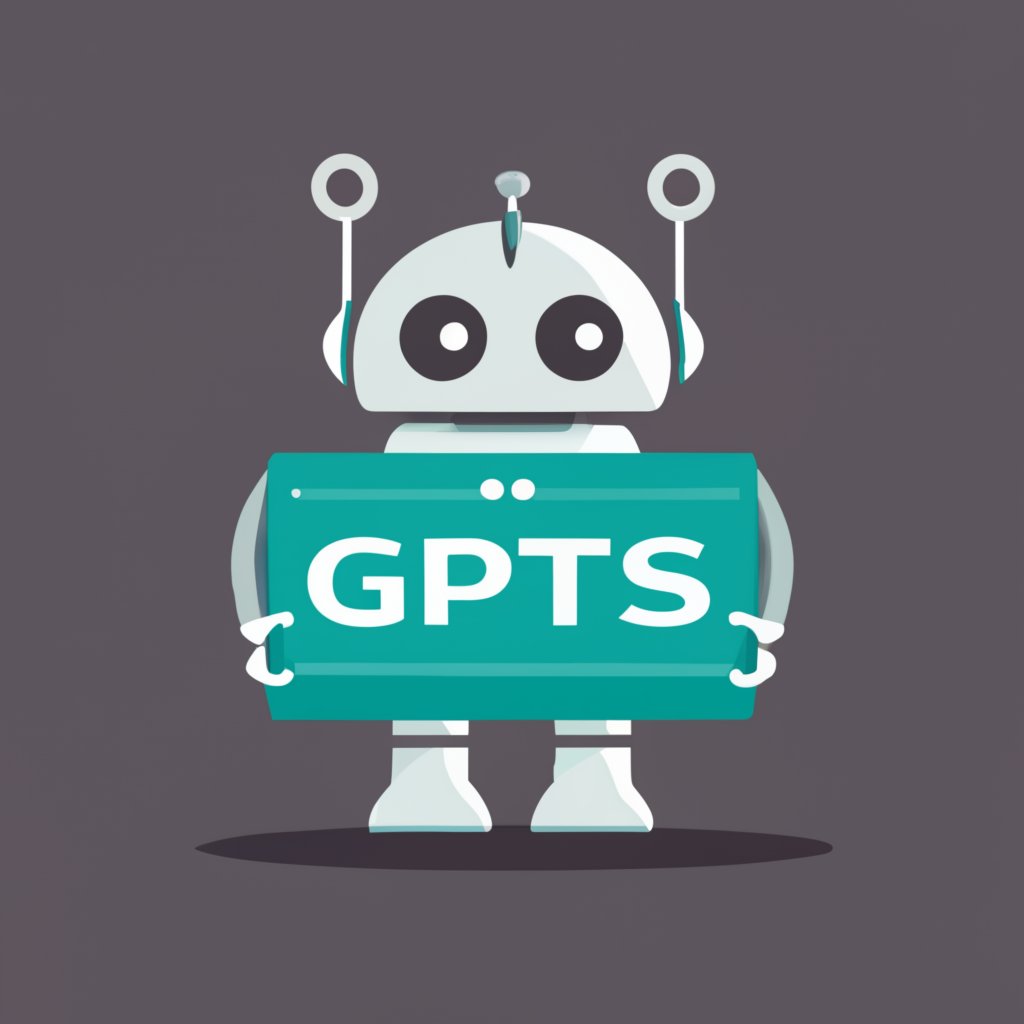
Chinese Assistant
AI-powered translations and summaries for Chinese.

Chinese Tutor
AI-powered translations and language learning
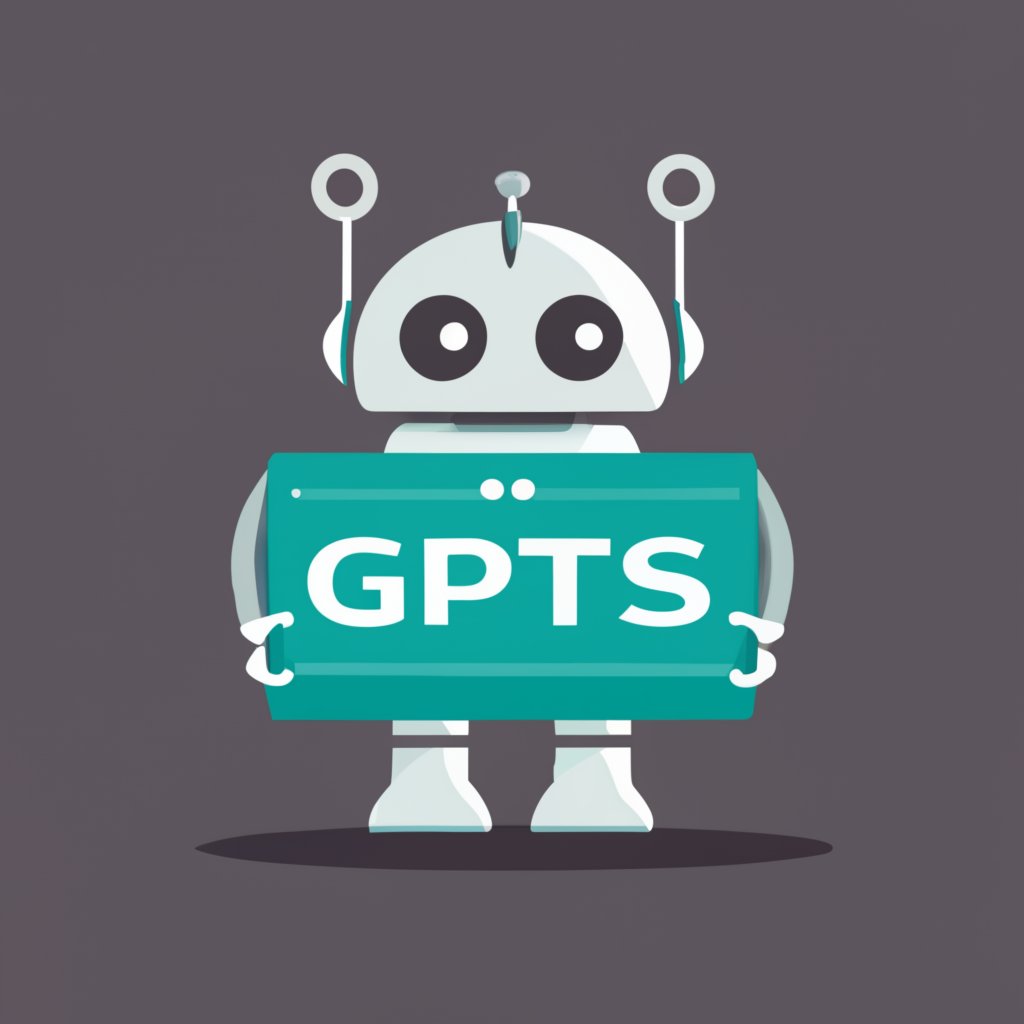
Critical Discourse
AI-powered debates for critical thinking

Audio Transcriber
AI-Driven Transcriptions Made Easy

- Data Analysis
- Hypothesis Testing
- Experiment Design
- Regression Analysis
- Multivariate Analysis
Detailed Q&A about Mathematical Statistics
What is the importance of the Cramer-Rao lower bound?
The Cramer-Rao lower bound provides a lower limit on the variance of unbiased estimators. It is a crucial concept because it helps determine the efficiency of an estimator. An estimator that achieves this bound is considered efficient and is the best unbiased estimator available.
How do you determine if an estimator is unbiased?
An estimator is unbiased if its expected value equals the true parameter value it is estimating. Mathematically, for an estimator T of a parameter θ, E[T] = θ. This property ensures that on average, the estimator hits the true parameter value.
What is Fisher information and why is it important?
Fisher information measures the amount of information that an observable random variable carries about an unknown parameter. It is important because it plays a key role in the Cramer-Rao bound, influencing the precision of parameter estimates.
What is a sufficient statistic?
A sufficient statistic is a function of the data that captures all the information needed to estimate a parameter. Formally, a statistic T(X) is sufficient for parameter θ if the conditional distribution of the data X given T(X) does not depend on θ.
How is hypothesis testing performed in statistical analysis?
Hypothesis testing involves formulating a null hypothesis (H0) and an alternative hypothesis (H1), selecting a significance level (α), computing a test statistic from the data, and then comparing this statistic to a critical value or using a p-value to decide whether to reject H0. Common tests include t-tests, chi-square tests, and ANOVA.