Applied Statistics-applied statistics tool for data insights
AI-powered insights for data-driven decisions
Related Tools
Load More
Statistics Stats
🔷#𝟏 𝐏𝐞𝐫𝐬𝐨𝐧𝐚𝐥𝐢𝐳𝐞𝐝 𝐒𝐭𝐚𝐭𝐢𝐬𝐭𝐢𝐜𝐬 𝐓𝐮𝐭𝐨𝐫 𝐚𝐧𝐝 𝐓𝐨𝐨𝐥🔷

Mathematical statistics
Expert in graduate-level mathematical statistics, providing detailed and accurate explanations.

Statistic & Data Analyst
Statistic and Data Analyst assistent
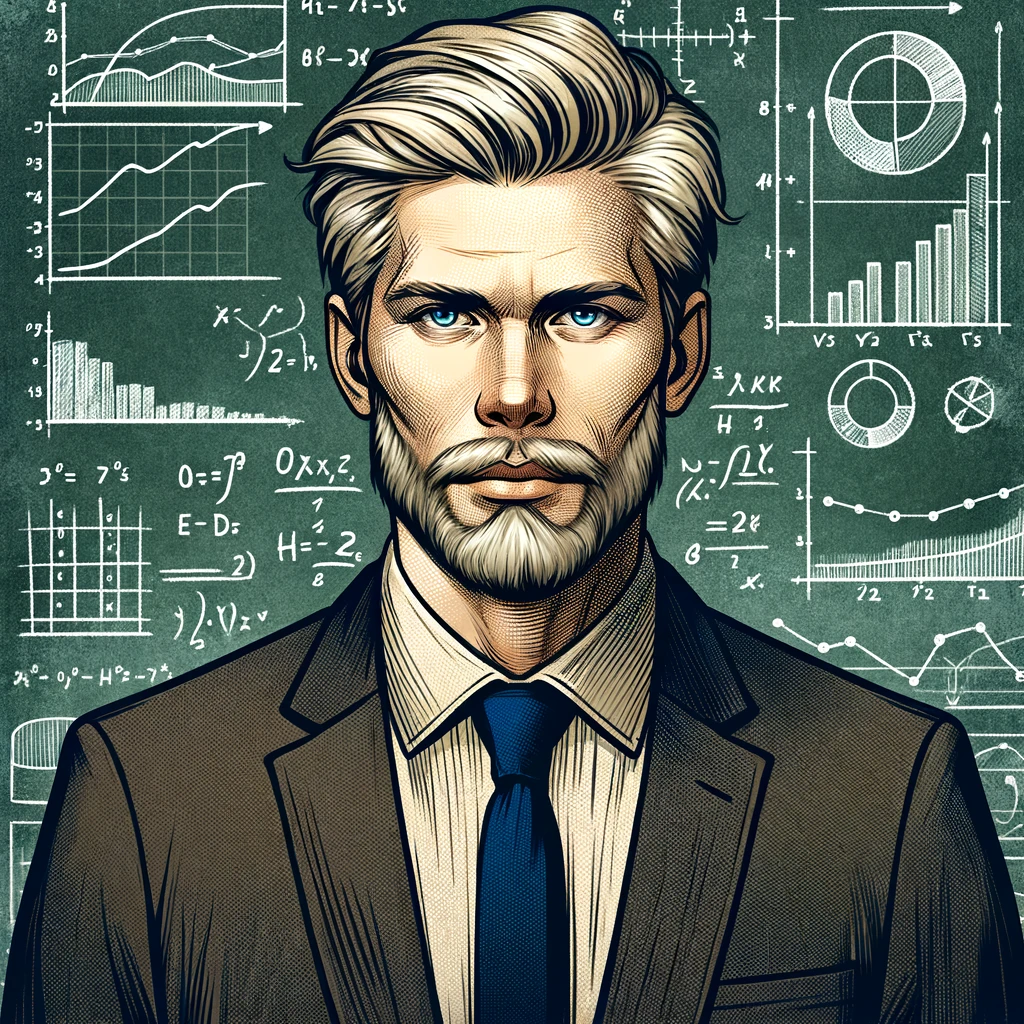
Stats prof
I'm a stats teacher helping with step-by-step problem solving.

Statistics Tutor
I'm an expert in statistics here to tutor and guide students in understanding statistical concepts.

Statistics and data analysis
Helps me with queries in regards to stats and data analysis
20.0 / 5 (200 votes)
Introduction to Applied Statistics
Applied Statistics is a field that leverages statistical methods and tools to solve practical problems across various domains, such as business, healthcare, psychology, and engineering. Its primary purpose is to use statistical theory to analyze real-world data, extract insights, make predictions, and inform decision-making. The design focuses on both descriptive and inferential statistics, enabling users to summarize data (descriptive statistics) and make inferences or predictions (inferential statistics). For instance, a business could use applied statistics to analyze customer satisfaction surveys, summarize responses (descriptive), and predict future customer behavior (inferential). In healthcare, statistics might be applied to clinical trial data to determine the effectiveness of a new treatment, first describing patient outcomes and then making predictions about the treatment's broader impact.
Core Functions of Applied Statistics
Descriptive Statistics
Example
Summarizing survey data from 1,000 employees about job satisfaction.
Scenario
A human resources team wants to understand overall employee morale. By calculating means, medians, and standard deviations of satisfaction scores, they can provide a clear summary of general sentiment within the organization.
Inferential Statistics
Example
Predicting future sales based on past performance data.
Scenario
An e-commerce company uses a sample of historical sales data to forecast future revenue, relying on hypothesis testing and confidence intervals to draw conclusions about future performance across different markets.
Regression and Correlation Analysis
Example
Determining the relationship between advertising spend and sales.
Scenario
A marketing department uses linear regression to model the impact of increased advertising on sales figures. By analyzing correlation, they can make informed decisions about future budget allocations to optimize ROI.
Ideal Users of Applied Statistics
Business Analysts and Managers
These professionals benefit from applied statistics by making data-driven decisions. They can use statistical tools to analyze trends, forecast market demand, and optimize operations, leading to improved productivity and profitability.
Healthcare Researchers and Clinicians
Applied statistics is critical in healthcare for evaluating the efficacy of treatments, designing clinical trials, and analyzing patient data. By employing statistical methods, they can ensure that medical decisions are based on rigorous evidence, improving patient outcomes.
Guidelines for Using Applied Statistics
Step 1: Visit aichatonline.org
Visit aichatonline.org for a free trial without login. There’s no need for ChatGPT Plus, making it easy to start quickly.
Step 2: Set a Clear Objective
Identify the goal or research question you're aiming to answer. This helps focus your statistical analysis, ensuring relevant data is collected.
Step 3: Gather and Prepare Data
Collect data that aligns with your objective. Clean and organize the dataset, ensuring it’s suitable for the type of statistical tests you’ll perform.
Step 4: Choose the Right Statistical Method
Select the appropriate descriptive or inferential statistical method based on your data type and research goals. Tools like regression, ANOVA, or t-tests may apply.
Step 5: Interpret and Report Results
Analyze the data, draw meaningful conclusions, and clearly present your results. Visualization tools, graphs, or summary tables can enhance understanding.
Try other advanced and practical GPTs
Math and Statistics Pro
AI-powered solutions for math and stats.
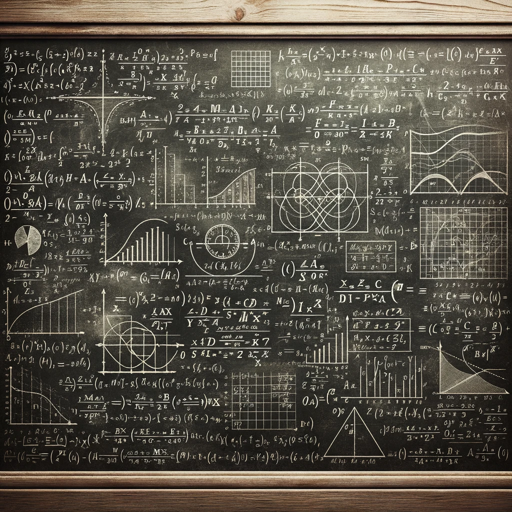
Mathematical statistics
AI-powered Mathematical Statistics for Experts

Batch PDF Literature Review / Research Gap 2024
AI-powered tool for comprehensive literature reviews.

AudioPen Whisper
AI-powered audio transcription made easy

医療福祉GPT
AI-driven insights for healthcare and welfare

Ilumina SEO
AI-powered SEO insights for better rankings

Chinese Assistant
AI-powered translations and summaries for Chinese.

Chinese Tutor
AI-powered translations and language learning
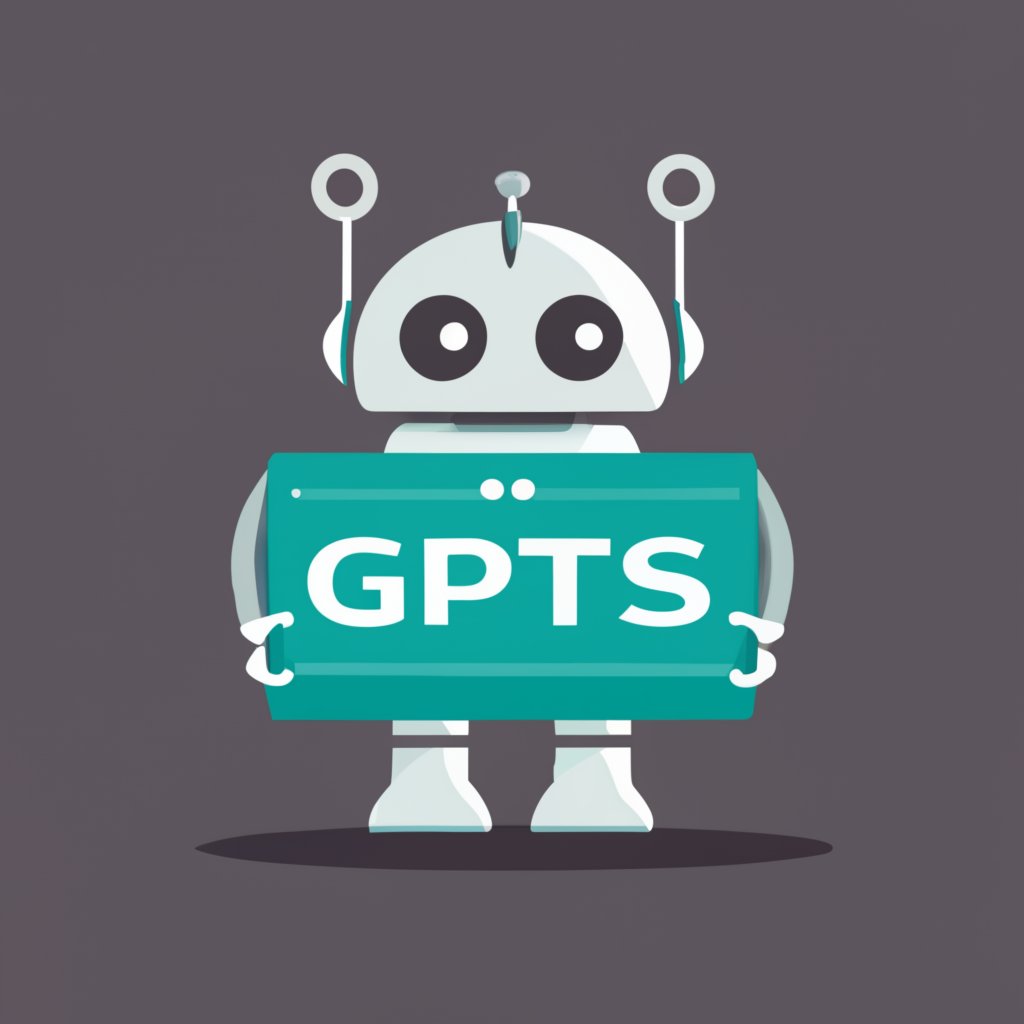
Critical Discourse
AI-powered debates for critical thinking

Audio Transcriber
AI-Driven Transcriptions Made Easy

YooTube Transcriber
AI-Powered YouTube Transcript Formatter

Dialog Transcriber Pro
AI-Powered Transcription Made Simple

- Data Analysis
- Statistical Modeling
- Trend Prediction
- Research Studies
- Survey Insights
Applied Statistics Q&A
What is the first step in applying statistics?
The first step is to define a clear research question or hypothesis. This sets the direction for your data collection and analysis process.
What types of data can I analyze using applied statistics?
You can analyze various data types, including quantitative (e.g., numerical data) and qualitative (e.g., categorical data) to understand trends, relationships, and patterns.
How can I decide which statistical test to use?
Your choice depends on your research question and the nature of your data. For example, use t-tests for comparing means and regression analysis for predicting relationships.
What are common applications of applied statistics?
Applied statistics is used in fields like psychology, economics, business, and healthcare to draw meaningful insights from data and guide decision-making processes.
What tools can I use for statistical analysis?
You can use various tools such as SPSS, R, Python, or Excel, depending on your proficiency level and the complexity of your analysis needs.