Discover Insights: Market Basket Analysis-AI-powered insights into market patterns
AI-Powered Insights for Market Basket Analysis

Analyze this market data to discover patterns: { 'Shirts': [1, 0, 1, 0, 1, 1, 0, 1], 'Pants': [0, 1, 0, 1, 0, 1, 1, 0], 'Shoes': [1, 1, 0, 0, 1, 0, 1, 1], 'Hats': [0, 0, 1, 0, 1, 1, 0, 1], 'Scarves': [1, 0, 1, 1, 0, 0, 0, 0] }
How should I send the data?
Based on the information above, how would you arrange the elements? { 'Novel': [1, 1, 0, 1, 0, 0, 1, 0], 'Science Fiction': [0, 1, 1, 0, 1, 0, 0, 1], 'Non-Fiction': [1, 0, 0, 0, 1, 1, 1, 1], 'Biography': [0, 0, 1, 1, 0, 1, 0, 0], 'Poetry': [0, 1, 0, 0, 1, 0, 1, 0] }
Interpret these association rules for marketing:
Related Tools
Load More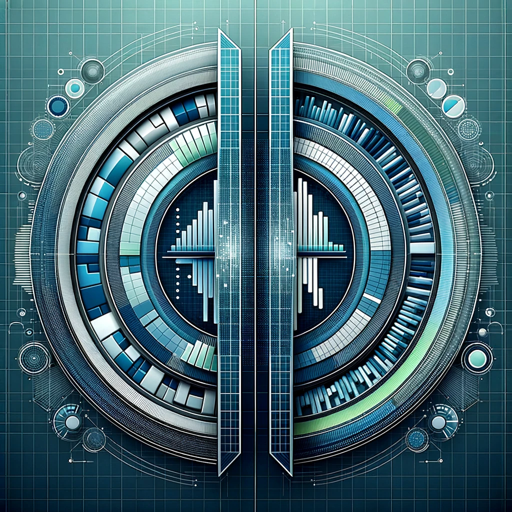
Data Science
Expert in data analysis and visualization.

Market Insight Analyst
Assists in conducting thorough industry analysis with research skills and tools.

A Data Analyzer
Expert in Data Analysis and Visualization

Data Insighter
Advanced data analyst, demystifying data with deeper insights.

Data Driven Marketing Strategy
Trasforma i dati disponibili online e offline in strategia di marketing

ON Marketing Insight
Especialista em Marketing, PNL e Análise de Dados
20.0 / 5 (200 votes)
Introduction to Discover Insights: Market Basket Analysis
Discover Insights: Market Basket Analysis is a specialized tool designed to analyze customer purchasing patterns using association rule mining and Boolean logic. Its primary function is to identify product correlations within large datasets, enabling businesses to optimize inventory management, develop targeted marketing strategies, and enhance the customer shopping experience. One of its core techniques is the Apriori algorithm, which efficiently discovers frequent itemsets and generates association rules such as 'If a customer buys bread, they are likely to buy milk.' In addition to traditional association rule mining, this tool also integrates Boolean logic to identify complex, non-linear relationships, such as exclusive or conditional patterns, providing a deeper understanding of consumer behavior. For example, a supermarket might learn that customers tend to buy cereal *or* milk, but rarely both together, indicating a need for differentiated promotions or shelf arrangements.
Main Functions of Discover Insights: Market Basket Analysis
Association Rule Mining
Example
Using the Apriori algorithm, this function identifies frequent patterns in customer transactions, revealing product pairs or combinations frequently purchased together. For instance, '80% of customers who buy pasta also purchase tomato sauce.'
Scenario
A grocery store chain can use this function to optimize its product placements, ensuring that commonly co-purchased items like pasta and sauce are displayed close together, increasing the likelihood of cross-selling.
Boolean Logic Analysis
Example
Through Boolean logic expressions such as AND, OR, XOR, the tool captures more complex relationships between items. For example, '(milk XOR cereal) AND (butter XOR bread)' indicates that customers buy one item from each category but rarely purchase both.
Scenario
This insight can be used by a retail store to design more nuanced promotions. If customers typically buy only one item in a category, it may be beneficial to create bundle deals that incentivize purchasing the complementary items.
Data-Driven Marketing Strategies
Example
By analyzing the confidence, support, and lift metrics of association rules, the tool helps identify high-impact products for targeted marketing. For example, '60% of customers who buy premium coffee also buy gourmet snacks.'
Scenario
This enables a specialty food retailer to create targeted campaigns promoting gourmet snacks to customers who have shown interest in premium coffee, boosting sales through personalized recommendations.
Ideal Users of Discover Insights: Market Basket Analysis
Retailers and Supermarkets
Retailers and supermarkets benefit significantly from the Market Basket Analysis tool as it helps them understand product affinities, optimize store layouts, and implement effective cross-selling strategies. By revealing which items are frequently purchased together, these businesses can improve product placement, reduce inventory costs, and tailor promotions based on customer buying behavior.
E-commerce Businesses
E-commerce platforms can leverage the tool to enhance their recommendation systems and personalize the online shopping experience. Understanding which products are likely to be purchased together allows e-commerce sites to create dynamic, targeted product suggestions, improving both user engagement and average order value.
How to Use Discover Insights: Market Basket Analysis
Visit aichatonline.org for a free trial without login, also no need for ChatGPT Plus.
The first step is to access the platform and explore the Market Basket Analysis tool for free without any registration.
Upload or Input Your Data
Use the provided interface to upload your transaction data in a suitable format, typically a CSV or JSON file containing binary or purchase history data.
Configure Analysis Settings
Select the type of analysis you'd like to run—Apriori, association rule mining, or Boolean logic minimization. Set support, confidence, or lift thresholds as necessary.
Run Analysis and Review Results
Start the analysis and review results such as frequent itemsets, association rules, or logical expressions. You can explore relationships between products or customer preferences.
Apply Insights for Business Strategy
Interpret the insights to improve marketing strategies, inventory management, and customer engagement by acting on discovered patterns and relationships.
Try other advanced and practical GPTs
Divorce Lawyer
AI-Powered Guidance for Divorce Proceedings

Child Book Story Maker
Create engaging children's stories with AI.

Math Step-by-Step
AI-powered math problem solver with step-by-step guidance.

Quote Companion
AI-powered quotes with visual flair

MS-SQL EXPERT
AI-powered T-SQL assistant

MS Office 365 Advisor
AI-powered guidance for Office 365

2how MJ Portrait
AI-powered prompt generation for photographers

AI Portrait Generator
AI-Powered Portrait Creation Made Easy

Quality Assurance Sidekick (QA)
AI-powered quality assurance and project management.
Photo Quality Enhancer
AI-Powered Image Enhancement Made Easy

Quality Management Excellence
AI-Driven Quality Management Solutions

Framework Wizard
AI-powered request structuring made easy

- Inventory Management
- Sales Forecasting
- Consumer Behavior
- Market Patterns
- Retail Analysis
Common Questions About Discover Insights: Market Basket Analysis
What is the purpose of Market Basket Analysis?
Market Basket Analysis helps identify relationships between products based on customer purchasing patterns, allowing businesses to discover which items are commonly bought together.
How does Discover Insights handle large datasets?
Discover Insights uses optimized algorithms like Apriori to efficiently process large datasets, ensuring quick analysis and generation of useful association rules and patterns.
Can I use this tool for non-retail data?
Yes, while Market Basket Analysis is popular in retail, it can be applied to any binary data for discovering patterns, such as website interactions, survey results, or healthcare datasets.
What is the difference between Apriori and Boolean logic analysis?
Apriori finds co-occurring items (association rules), while Boolean logic focuses on exclusive relationships or patterns of mutually exclusive behaviors in customer purchasing habits.
What data format is required for analysis?
The tool requires a binary matrix or transactional data in formats like CSV or JSON, where rows represent transactions and columns represent items.