Data Analytics in Business Process Version 1.2-Data-Driven Business Insights
AI-powered analytics for process optimization

How to use & capability ?
What's chatbot update?
Related Tools
Load More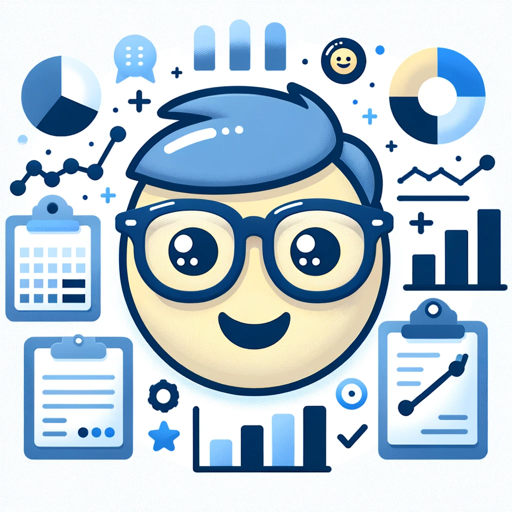
Data Analytics
A how-to guide for data analytics (based on Luke Barousse's 'ChatGPT for Data Analytics' course)

Advanced Data Analysis
Advanced data analysis assistant offering insights and guidance.
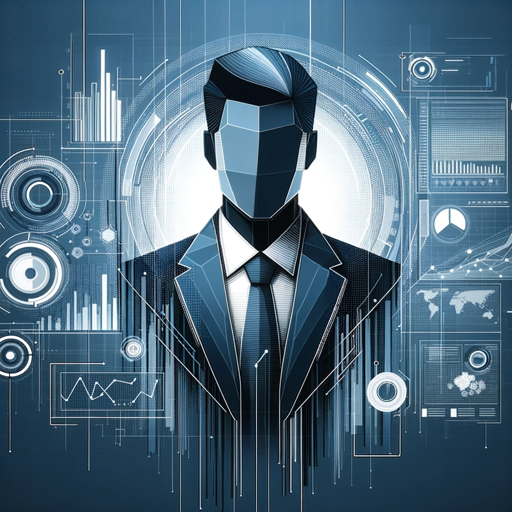
Business Analyst
Expert in business analysis and documentation.
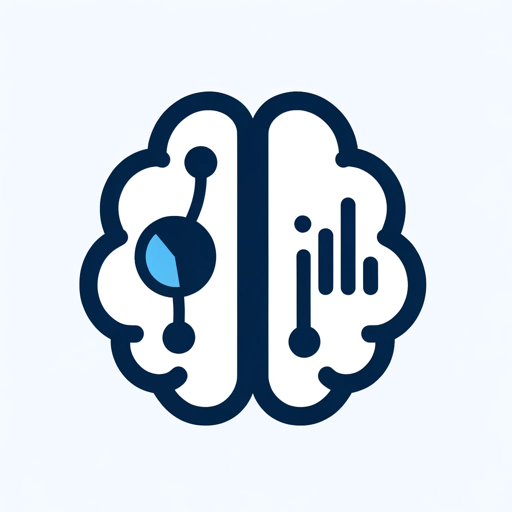
GPT Business Intelligence
Expert in Business Data Analysis for Strategic Insights
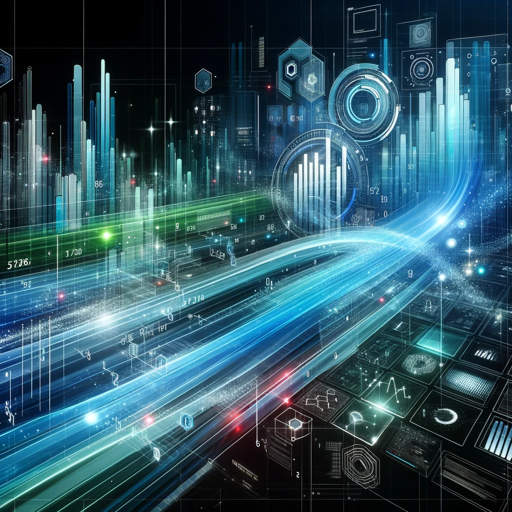
Advanced Big Data Analytics
Advanced big data analysis tool for insightful patterns, trends, and predictions.

Analytics Expert
Expert in Google Analytics 4, GTM, BigQuery, and Looker Studio
20.0 / 5 (200 votes)
Introduction to Data Analytics in Business Process Version 1.2
Data Analytics in Business Process Version 1.2 is designed to provide a specialized analytical framework tailored for business processes, with a focus on data-driven decision-making. The core purpose is to help organizations improve their operational efficiency and strategic outcomes through advanced analytics techniques. For instance, in the banking industry, this version is particularly useful for enhancing the debt collection process by integrating data analytics with Business Process Reengineering (BPR) to optimize risk management and operational performance. Version 1.2 refines the analytics model framework to be more applicable across different business scenarios, ensuring that users can adapt it to their specific needs, whether it be in resource allocation, workload forecasting, or customer behavior analysis.
Main Functions of Data Analytics in Business Process Version 1.2
Analytics Model Framework (AMF)
Example
The AMF can be applied to the mortgage services process, where it breaks down the process into four models: Application and Approval, Processing and Underwriting, Closing and Customer Onboarding, and Servicing and Portfolio Management.
Scenario
In a mortgage service, AMF helps in assessing eligibility and risk during the application phase, verifying information in underwriting, ensuring smooth closing, and managing ongoing customer relationships. For example, predictive analytics within the AMF can forecast the likelihood of loan approval based on applicant profiles, helping banks make informed decisions.
Analytics Hypothesis Generation
Example
A hypothesis could be generated around optimizing resource allocation in debt collection by analyzing the relationship between customer segmentation and default probability.
Scenario
In the context of debt collection, generating a hypothesis like 'Does allocating cases based on customer segmentation and default probability improve success rates?' allows for testing and refining collection strategies using data-driven insights.
Mock Data Creation
Example
Mock data can be created for roll rate analysis in debt collections, including variables such as customer ID, delinquency status, and payment history.
Scenario
For a debt collection team preparing a Power BI dashboard, mock data can be generated to simulate various scenarios, such as the impact of different collection strategies on roll rates, allowing the team to visualize potential outcomes before implementing changes.
Ideal Users of Data Analytics in Business Process Version 1.2
Banking and Financial Services Professionals
This group includes credit risk managers, debt collection teams, and business analysts who need to optimize processes such as loan approvals, customer onboarding, and debt collection. They benefit from the detailed analytics models and mock data capabilities, which help in making informed decisions and improving operational efficiency.
Business Process Reengineering (BPR) Teams
These users are involved in redesigning business processes to improve efficiency and effectiveness. By leveraging the analytics tools provided in Version 1.2, they can integrate data analytics into their process improvements, particularly in areas like resource allocation, workflow optimization, and customer segmentation.
How to Use Data Analytics in Business Process Version 1.2
Step 1
Visit aichatonline.org to start a free trial without needing to log in or have a ChatGPT Plus subscription.
Step 2
Familiarize yourself with the specific use cases and capabilities of Data Analytics in Business Process Version 1.2, including understanding key terms and framework models like ABC.
Step 3
Identify the data sources and analytics maturity levels needed for your business process, utilizing tools such as RPA and workflow automation for streamlining operations.
Step 4
Leverage analytics techniques at the descriptive, diagnostic, predictive, and prescriptive levels to gain insights and make informed decisions.
Step 5
Implement and monitor the results, continuously refining your approach based on feedback and new data, ensuring alignment with business goals and compliance standards like IFRS 9 and TFRS 9.
Try other advanced and practical GPTs
Image Copy Machine 👉🏼 Auto-improve version
AI-powered image enhancement and merging
dungeons and dragons DnD
AI-Powered Dungeon Master for Epic Adventures

Auto Expert
AI-powered solutions for automotive excellence.

Christian Prayer - Bible CoPilot
Empowering Your Prayer Life with AI

Your Favorite Attending
AI-powered clinical decisions, simplified.

Electronics and Circuit Analysis
AI-powered Circuit Analysis and Design

Practical Version: OPNS451 Quiz-Prep Tutor
Turn sketches into R code effortlessly.

Live
AI-powered solutions for every task.
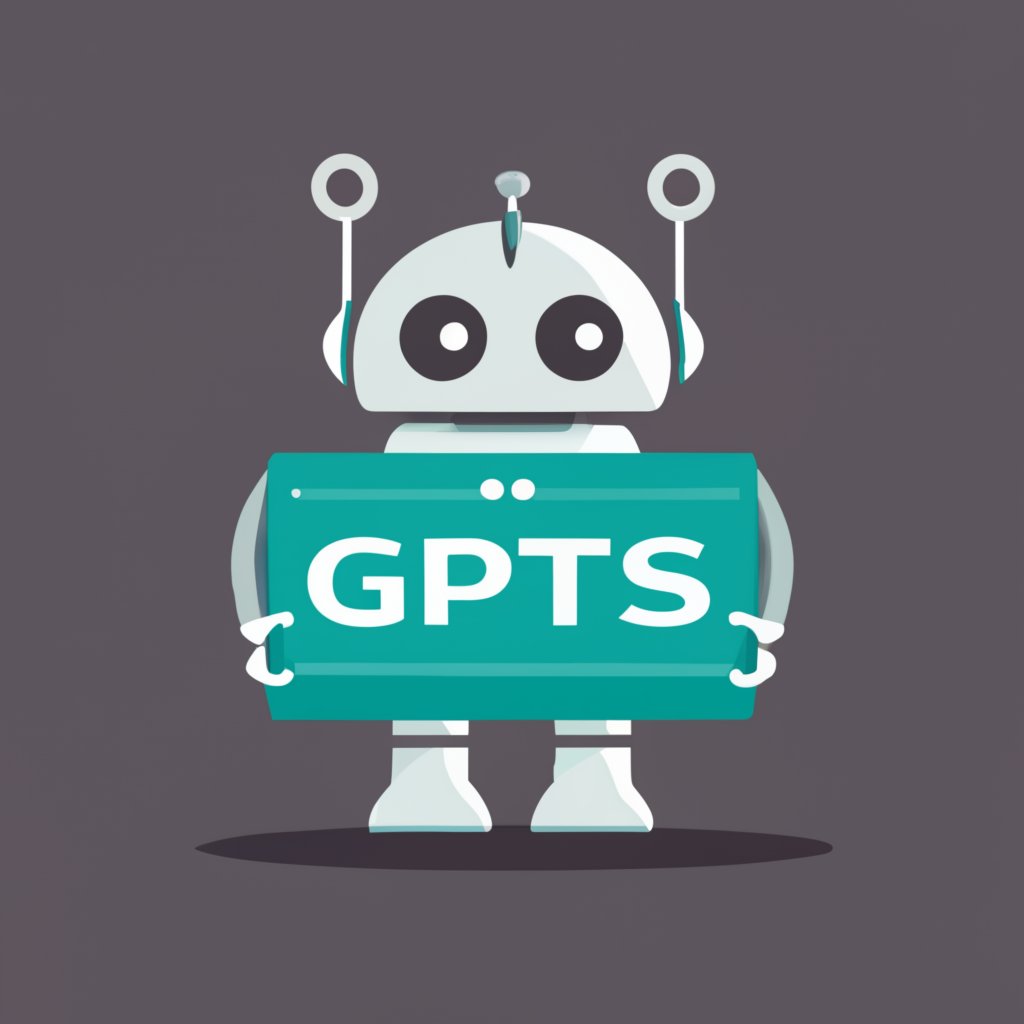
European Patent Attorney & Litigator
AI-powered tool for patent law expertise.

Bender
AI with bite, no fluff.

Explore Philosophy (Philosophy Tutor)
AI-powered insights into philosophy.

No Slang
Refine casual language with AI precision.

- Risk Management
- Data Visualization
- Predictive Modeling
- Process Automation
- Compliance Reporting
Q&A on Data Analytics in Business Process Version 1.2
What is the main purpose of Data Analytics in Business Process Version 1.2?
The main purpose is to enhance business process efficiency and decision-making through data-driven insights, particularly in the context of banking collection processes.
How does the ABC model framework integrate with data analytics?
The ABC model framework segments the collection process into Approval, Behavior, and Collection, providing a structured approach for applying descriptive, diagnostic, predictive, and prescriptive analytics.
Can Data Analytics in Business Process Version 1.2 help with risk management?
Yes, it aids in assessing and managing credit risk, especially by utilizing predictive analytics to forecast potential defaults and optimize the collection strategy.
What technologies are essential for implementing this version of data analytics in business processes?
Key technologies include Robotic Process Automation (RPA), Workflow Automation, and Business Intelligence tools such as Power BI and Tableau.
How does Data Analytics in Business Process Version 1.2 support compliance with financial reporting standards?
The framework ensures that analytics models align with IFRS 9 and TFRS 9 standards, aiding in the accurate assessment of financial risk and appropriate reporting.